Documents
Presentation Slides
Two-Step Sound Source Separation: Training on Learned Latent Targets (Presentation)
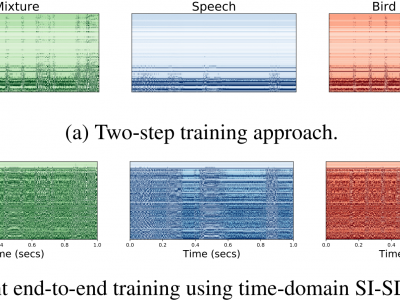
- Citation Author(s):
- Submitted by:
- Efthymios Tzinis
- Last updated:
- 20 April 2020 - 7:15pm
- Document Type:
- Presentation Slides
- Document Year:
- 2020
- Event:
- Presenters:
- Efthymios Tzinis
- Paper Code:
- AUD-L2.1
- Categories:
- Log in to post comments
In this paper, we propose a two-step training procedure for source separation via a deep neural network. In the first step we learn a transform (and it's inverse) to a latent space where masking-based separation performance using oracles is optimal. For the second step, we train a separation module that operates on the previously learned space. In order to do so, we also make use of a scale-invariant signal to distortion ratio (SI-SDR) loss function that works in the latent space, and we prove that it lower-bounds the SI-SDR in the time domain. We run various sound separation experiments that show how this approach can obtain better performance as compared to systems that learn the transform and the separation module jointly. The proposed methodology is general enough to be applicable to a large class of neural network end-to-end separation systems.