Documents
Presentation Slides
Unconstrained Periocular Recognition: Using Generative Deep Learning Frameworks for Attribute Normalization
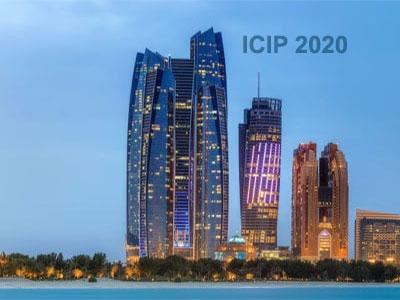
- Citation Author(s):
- Submitted by:
- Luiz Zanlorensi
- Last updated:
- 3 November 2020 - 5:46pm
- Document Type:
- Presentation Slides
- Document Year:
- 2020
- Event:
- Presenters:
- Luiz A. Zanlorensi
- Paper Code:
- 2644
- Categories:
- Log in to post comments
Ocular biometric systems working in unconstrained environments usually face the problem of small within-class compactness caused by the multiple factors that jointly degrade the quality of the obtained data. In this work, we propose an attribute normalization strategy based on deep learning generative frameworks, that reduces the variability of the samples used in pairwise comparisons, without reducing their discriminability. The proposed method can be seen as a preprocessing step that contributes for data regularization and improves the recognition accuracy, being fully agnostic to the recognition strategy used. As proof of concept, we consider the “eyeglasses” and “gaze” factors, comparing the levels of performance of five different recognition methods with/without using the proposed normalization strategy. Also, we introduce a new dataset for unconstrained periocular recognition, composed of images acquired by mobile devices, particularly suited to perceive the impact of “wearing eyeglasses” in recognition effectiveness. Our experiments were performed in two different datasets, and support the usefulness of our attribute normalization scheme to improve the recognition performance.