Documents
Poster
UNSUPERVISED CROSS-CORPUS SPEECH EMOTION RECOGNITION USING DOMAIN-ADAPTIVE SUBSPACE LEARNING
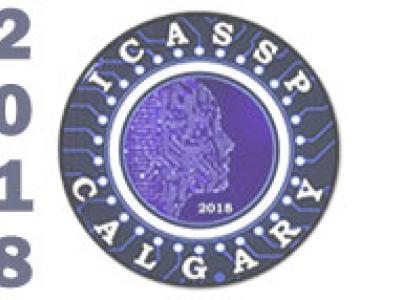
- Citation Author(s):
- Submitted by:
- Na Liu
- Last updated:
- 13 April 2018 - 8:10am
- Document Type:
- Poster
- Document Year:
- 2018
- Event:
- Presenters:
- Na Liu
- Paper Code:
- 2600
- Categories:
- Log in to post comments
In this paper, we investigate an interesting problem, i.e., unsupervised cross-corpus speech emotion recognition (SER), in which the training and testing speech signals come from two different speech emotion corpora. Meanwhile, the training speech signals are labeled, while the label information of the testing speech signals is entirely unknown. Due to this setting, the training (source) and testing (target) speech signals may have different feature distributions and therefore lots of existing SER methods would not work. To deal with this problem, we propose a domain-adaptive subspace learning (DoSL) method for learning a projection matrix with which we can transform the source and target speech signals from the original feature space to the label space. The transformed source and target speech signals in the label space would have similar feature distributions. Consequently, the classifier learned on the labeled source speech signals can effectively predict the emotional states of the unlabeled target speech signals. To evaluate the performance of the proposed DoSL method, we carry out extensive cross-corpus SER experiments on three speech emotion corpora including EmoDB, eNTERFACE, and AFEW 4.0. Compared with recent state-of-the-art cross-corpus SER methods, the proposed DoSL can achieve more satisfactory overall results.