Documents
Presentation Slides
Unsupervised Speaker Adaptation of BLSTM-RNN for LVCSR Based on Speaker Code
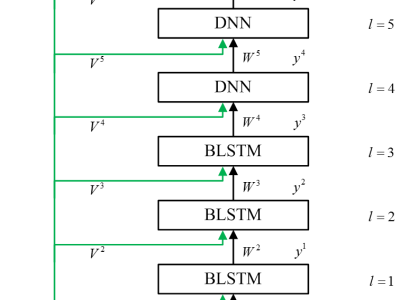
- Citation Author(s):
- Submitted by:
- Zhiying Huang
- Last updated:
- 14 October 2016 - 10:15am
- Document Type:
- Presentation Slides
- Document Year:
- 2016
- Event:
- Presenters:
- Zhiying Huang
- Paper Code:
- ISCLSP-O11-1
- Categories:
- Log in to post comments
Recently, the speaker code based adaptation has been successfully expanded to recurrent neural networks using bidirectional Long Short-Term Memory (BLSTM-RNN) [1]. Experiments on the small-scale TIMIT task have demonstrated that the speaker code based adaptation is also valid for BLSTM-RNN. In this paper, we evaluate this method on large-scale task and introduce an error normalization method to balance the back-propagation errors derived from different layers for speaker codes. Meanwhile, we use singular value decomposition (SVD) method to conduct model compression. Results show that the speaker code based adaptation with SVD shows better recognition performance than the i-vector based speaker adaptation of the same dimension. Experimental results on Switchboard task show that the speaker code based adaptation on the hybrid BLSTM-DNN topology can achieve more than 9% relative reduction in word error rate (WER) compared to the speaker independent (SI) baseline.