Documents
Presentation Slides
VISUALIZING NETWORK CONNECTIVITY IN PARKINSON’S DISEASE
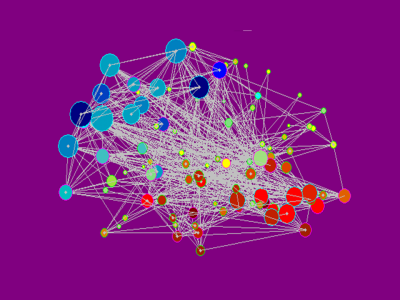
- Citation Author(s):
- Submitted by:
- Phoebe Spetsieris
- Last updated:
- 7 October 2018 - 11:56pm
- Document Type:
- Presentation Slides
- Document Year:
- 2018
- Event:
- Presenters:
- Phoebe Spetsieris
- Paper Code:
- WA.L3.3
- Categories:
- Log in to post comments
Numerous recent papers have demonstrated the utility of graph theoretical analysis in conjunction with sparse inverse covariance estimation (SICE) in understanding the modulation of brain connectivity associated with neuropathology. These concepts may complement established knowledge of functional covariance obtained using principal component analysis (PCA) that can reduce whole data representations of brain data to essential disease specific patterns. The potential informative advantage of joint SICE/PCA applicability is demonstrated here in the study and diagnosis of Parkinson’s disease (PD) in metabolic fluorodeoxyglucose ([18F]FDG) positron emission tomography (PET) data. The relationship of the two reduction techniques is demonstrated and the graphical parameters of the SICE representation are deduced in a novel processing step and applied to study and visualize the underlying functional connectivity of the homologous PC imaging biomarker.