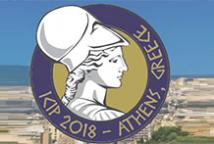
The International Conference on Image Processing (ICIP), sponsored by the IEEE Signal Processing Society, is the premier forum for the presentation of technological advances and research results in the fields of theoretical, experimental, and applied image and video processing. ICIP has been held annually since 1994, brings together leading engineers and scientists in image and video processing from around the world. Visit website.
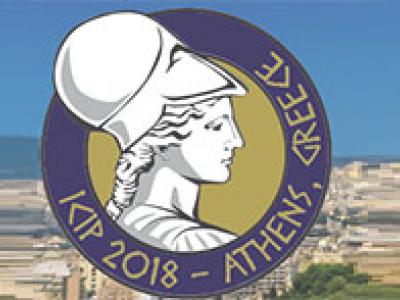
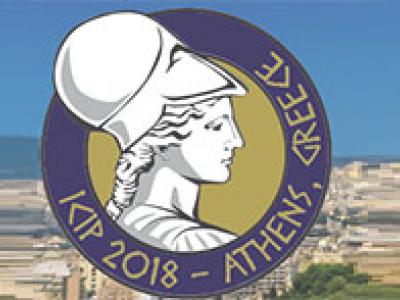
- Read more about Distortion-Robust Spherical Camera Motion Estimation via Dense Optical Flow
- Log in to post comments
Conventional techniques for frame-to-frame camera motion estimation rely on tracking a set of sparse feature points. However, images taken from spherical cameras have high distortion which can induce mistakes in feature point tracking, offsetting the advantage of their large fields-of-view. Hence, in this research, we attempt a novel approach of using dense optical flow for distortion-robust spherical camera motion estimation. Dense optical flow incorporates smoothing terms and is free of local outliers. It encodes the camera motion as well as dense 3D information.
- Categories:
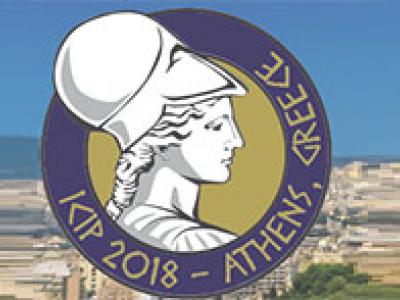
- Read more about Recurrent and Dynamic Models for Predicting Streaming Video Quality of Experience
- Log in to post comments
- Categories:
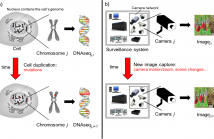
- Read more about Profile Hidden Markov Models for Foreground Object Modelling
- Log in to post comments
Accurate background/foreground segmentation is a preliminary process essential to most visual surveillance applications. With the increasing use of freely moving cameras, strategies have been proposed to refine initial segmentation. In this paper, it is proposed to exploit the Vide-omics paradigm, and Profile Hidden Markov Models in particular, to create a new type of object descriptors relying on spatiotemporal information. Performance of the proposed methodology has been evaluated using a standard dataset of videos captured by moving cameras.
- Categories:
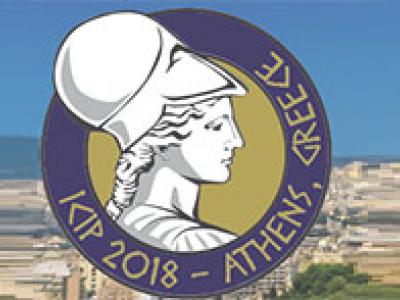
- Read more about Code: LOW-FREQUENCY IMAGE NOISE REMOVAL USING WHITE NOISE FILTER
- Log in to post comments
Image noise filters usually assume noise as white Gaussian. However, in a capturing pipeline, noise often becomes spatially correlated due to in-camera processing that aims to suppress the noise and increase the compression rate. Mostly, only high-frequency noise components are suppressed since the image signal is more likely to appear in the low-frequency components of the captured image. As a result, noise emerges as coarse grain which makes white (all-pass) noise filters ineffective, especially when the resolution of the target display is lower than the captured image.
- Categories:
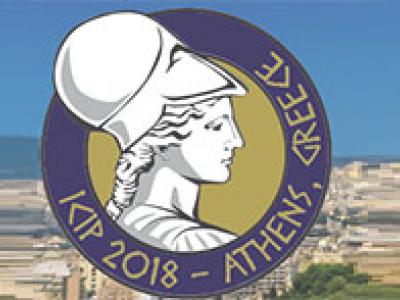
- Read more about SEGMENTATION OF LUNG TUMOR IN CONE BEAM CT IMAGES BASED ON LEVEL-SETS
- Log in to post comments
poster_v2.pdf

- Categories:
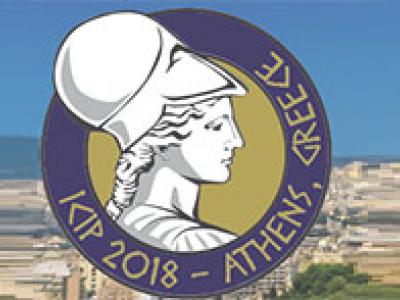
- Read more about A UNIFIED FRAMEWORK FOR FAULT DETECTION OF FREIGHT TRAIN IMAGES UNDER COMPLEX ENVIRONMENT
- Log in to post comments
This paper proposes a novel unified framework for fault detection of the freight train images based on convolutional neural network (CNN) under complex environment. Firstly, the multi region proposal networks (MRPN) with a set of prior bounding boxes are introduced to achieve high quality fault proposal generation. And then, we apply a linear non-maximum suppression method to retain the most suitable anchor while removing redundant boxes. Finally, a powerful multi-level region-of-interest (ROI) pooling is proposed for proposal classification and accurate detection.
- Categories:
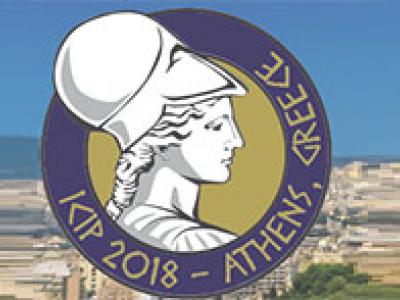
- Read more about On Regression Losses for Depth Estimation
- Log in to post comments
Depth estimation from a single monocular image has reached great performances thanks to recent works based on deep networks. However, as various choices of losses, architectures and experimental conditions are proposed in the literature, it is difficult to establish their respective influence on the performances. In this paper we propose an in-depth study of various losses and experimental conditions for depth regression, on \nyu dataset. From this study we propose a new network for depth estimation combining an encoder-decoder architecture with an adversarial loss.
- Categories:
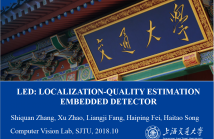
Classification subnetwork and box regression subnetwork are
essential components in deep networks for object detection.
However, we observe a contradiction that before NMS, some
better localized detections do not correspond to higher classification confidences, and vice versa. This contradiction exists because classification confidences can not fully reflect the
localization-quality (loc-quality) of each detection. In this
work, we propose the Localization-quality Estimation embedded Detector abbreviated as LED, and a corresponding
- Categories: