Documents
Supplementary Material
WaveE2VID: FREQUENCY-AWARE EVENT-BASED VIDEO RECONSTRUCTION
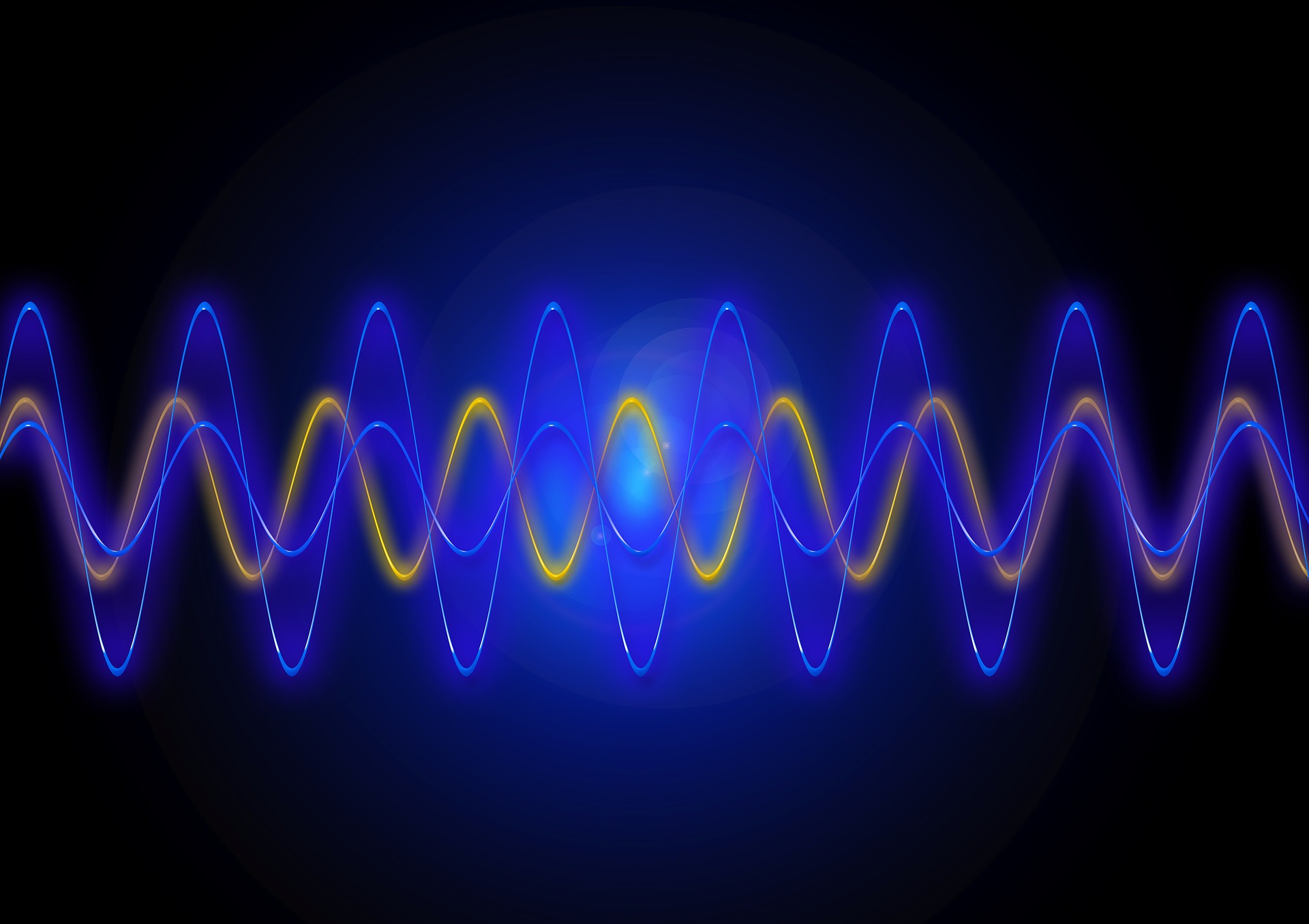
- DOI:
- 10.60864/1tcr-tt30
- Citation Author(s):
- Submitted by:
- Anonymous author
- Last updated:
- 1 February 2025 - 12:15pm
- Document Type:
- Supplementary Material
- Document Year:
- 2025
- Presenters:
- Anonymous
- Categories:
- Log in to post comments
Event cameras, which detect local brightness changes instead of capturing full-frame images, offer high temporal resolution and low latency. Although existing convolutional neural networks (CNNs) and transformer-based methods for event-based video reconstruction have achieved impressive results, they suffer from high computational costs due to their linear operations. These methods often require 10M-30M parameters and inference times of 30-110 ms per forward pass at a resolution of 640\times480 on modern GPUs. Furthermore, to reduce computational costs, these methods apply CNN-based downsampling, which leads to the loss of fine details. To address these challenges, we propose an efficient hybrid model, WaveE2VID, which combines the frequency-domain analysis of the wavelet transform with the spatio-temporal context modeling of a deep convolutional recurrent network. Our model achieves 50% faster inference speed and lower GPU memory usage than CNN and transformer-based methods, maintaining reconstruction performance on par with state-of-the-art approaches across benchmark datasets