Documents
Poster
Hierarchical Activity Clustering Analysis for Robust Graphical Structure Recovery
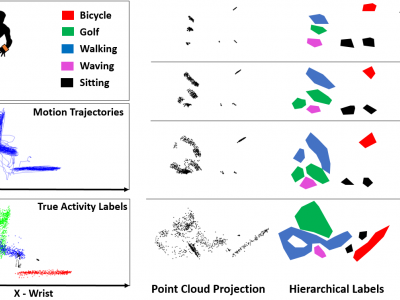
- Citation Author(s):
- Submitted by:
- Namita Lokare
- Last updated:
- 26 November 2016 - 11:17am
- Document Type:
- Poster
- Document Year:
- 2016
- Event:
- Presenters:
- Namita Lokare
- Paper Code:
- 1371
- Categories:
- Keywords:
- Log in to post comments
In this paper we propose a hierarchical activity clustering methodology which incorporates the use of topological persistence analysis. Our clustering methodology captures the hierarchies present in the data and is therefore able to show the dependencies that exist between these activities. We make use of an aggregate persistence diagram to select robust graphical structures present within the dataset. These models are stable over a bound and provide accurate classification results. This approach allows us to select parameters based on the amount of temporal information needed to maintain high accuracy. The key innovations presented in this paper include the hierarchical characterization of the activities over a temporal parameter as well as the characterization and parameter selection based on stability of the results using persistence analysis.