Documents
Poster
Minimum-Volume Weighted Symmetric Nonnegative Matrix Factorization for Clustering
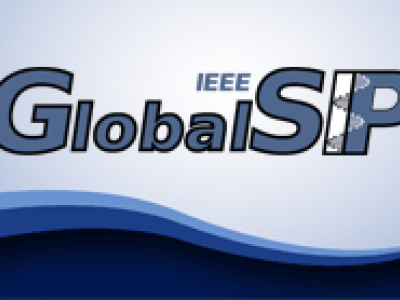
- Citation Author(s):
- Submitted by:
- Tianxiang Gao
- Last updated:
- 6 December 2016 - 7:16pm
- Document Type:
- Poster
- Event:
- Presenters:
- Tianxiang Gao
- Paper Code:
- 1168
- Categories:
- Log in to post comments
In recent years, nonnegative matrix factorization (NMF) attracts much attention in machine learning and signal processing fields due to its interpretability of data in a low dimensional subspace. For clustering problems, symmetric nonnegative matrix factorization (SNMF) as an extension of NMF factorizes the similarity matrix of data points directly and outperforms NMF when dealing with nonlinear data structure. However, the clustering results of SNMF is very sensitive to noisy data. In this paper, we propose a minimum-volume weighted SNMF (MV-WSNMF) based on the relationship between robust NMF and SNMF. The proposed MV-WSNMF can approximate the similarity matrices flexibly such that the resulting performance is more robust against noise. A computationally efficient algorithm is also proposed with convergence guarantee. The numerical simulation results show the improvement of the pro- posed algorithm with respective to clustering accuracy in comparison with the state-of-the-art algorithms.