Documents
Presentation Slides
On Modular Training of Neural Acoustics-to-Word Model for LVCSR
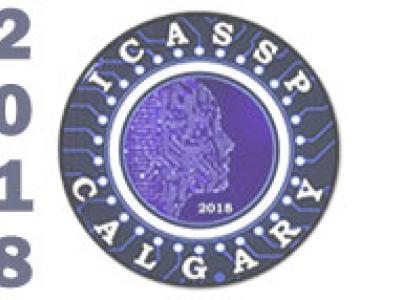
- Citation Author(s):
- Submitted by:
- Zhehuai Chen
- Last updated:
- 12 April 2018 - 12:34pm
- Document Type:
- Presentation Slides
- Document Year:
- 2018
- Event:
- Presenters:
- Zhehuai Chen
- Paper Code:
- SP-L1.2
- Categories:
- Log in to post comments
End-to-end (E2E) automatic speech recognition (ASR) systems directly map acoustics to words using a unified model. Previous works
mostly focus on E2E training a single model which integrates acoustic and language model into a whole. Although E2E training benefits
from sequence modeling and simplified decoding pipelines, large
amount of transcribed acoustic data is usually required, and traditional acoustic and language modelling techniques cannot be utilized. In this paper, a novel modular training framework of E2E ASR
is proposed to separately train neural acoustic and language models
during training stage, while still performing end-to-end inference in
decoding stage. Here, an acoustics-to-phoneme model (A2P) and a
phoneme-to-word model (P2W) are trained using acoustic data and
text data respectively. A phone synchronous decoding (PSD) module
is inserted between A2P and P2W to reduce sequence lengths without precision loss. Finally, modules are integrated into an acousticsto-word model (A2W) and jointly optimized using acoustic data to
retain the advantage of sequence modeling. Experiments on a 300-
hour Switchboard task show significant improvement over the direct
A2W model. The efficiency in both training and decoding also benefits from the proposed method.