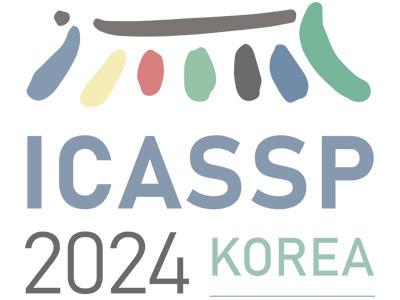
- Read more about Investigating End-to-end ASR Architectures for Long form Audio Transcription
- Log in to post comments
This paper presents an overview and evaluation of some of the end-to-end ASR models on long-form audios. We study three categories of Automatic Speech Recognition(ASR) models based on their core architecture: (1) convolutional, (2) convolutional with squeeze-and-excitation and (3) convolutional models with attention. We selected one ASR model from each category and evaluated Word Error Rate, maximum audio length and real-time factor for each model on a variety of long audio benchmarks: Earnings-21 and 22, CORAAL, and TED-LIUM3.
- Categories:
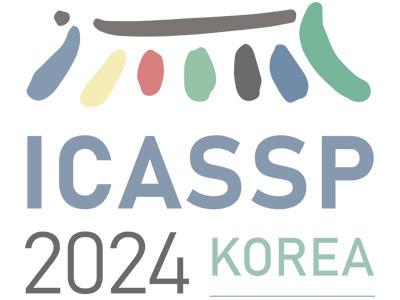
- Read more about Less peaky and more accurate CTC forced alignment by label priors
- 1 comment
- Log in to post comments
Connectionist temporal classification (CTC) models are known to have peaky output distributions. Such behavior is not a problem for automatic speech recognition (ASR), but it can cause inaccurate forced alignments (FA), especially at finer granularity, e.g., phoneme level. This paper aims at alleviating the peaky behavior for CTC and improve its suitability for forced alignment generation, by leveraging label priors, so that the scores of alignment paths containing fewer blanks are boosted and maximized during training.
aligner.pdf

- Categories:
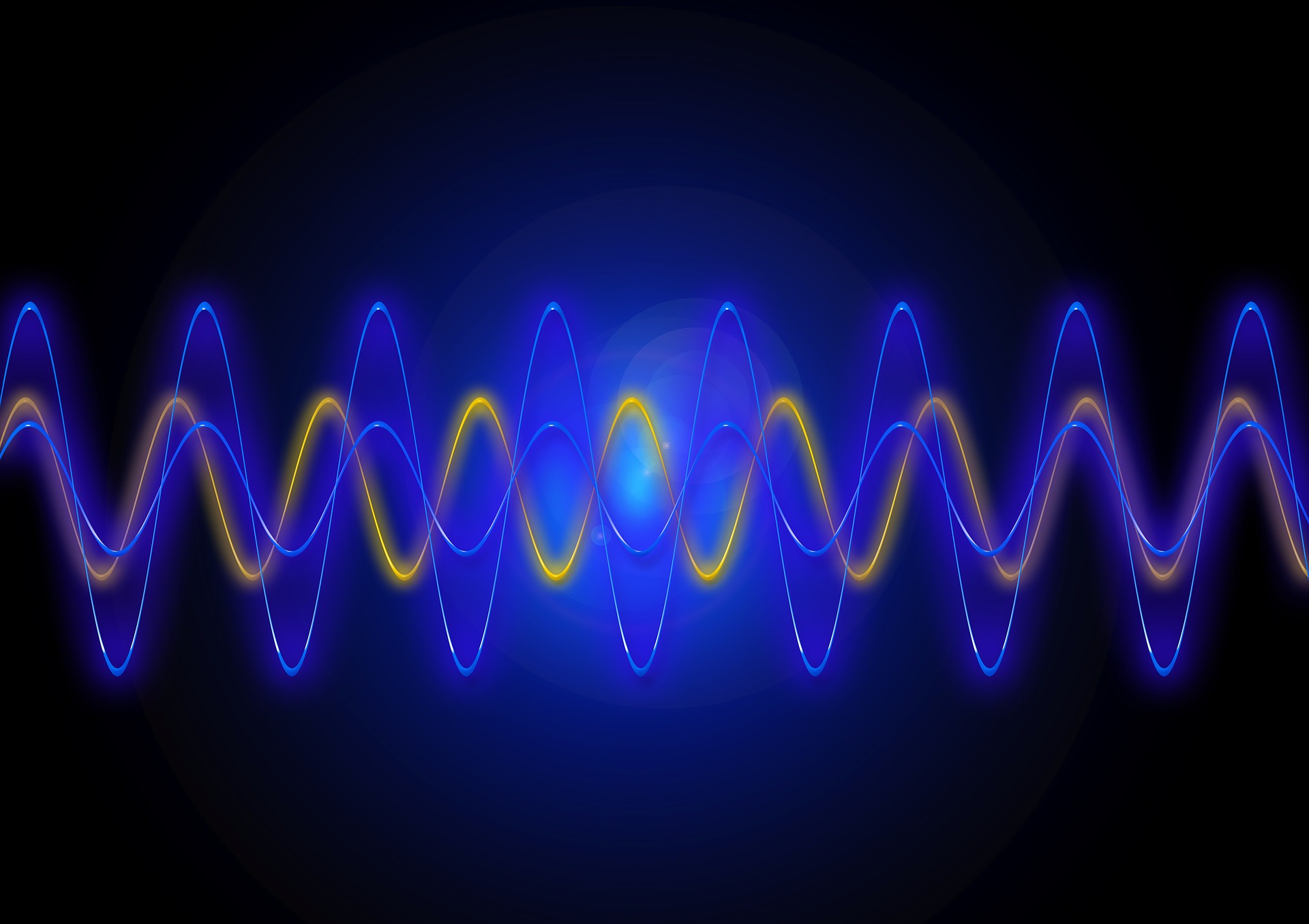
This report describes the submitted system to the In-Car Multi-Channel Automatic Speech Recognition (ICMC-ASR) challenge, which considers the ASR task with multi-speaker overlapping and Mandarin accent dynamics in the ICMC case. We implement the front-end speaker diarization using the self-supervised learning representation based multi-speaker embedding and beamforming using the speaker position, respectively. For ASR, we employ an iterative pseudo-label generation method based on fusion model to obtain text labels of unsupervised data.
- Categories:
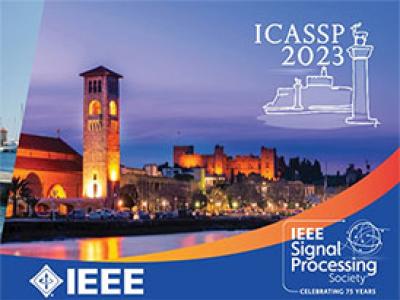
- Read more about DYNAMIC ALIGNMENT MASK CTC: IMPROVED MASK CTC WITH ALIGNED CROSS ENTROPY
- Log in to post comments
- Categories:
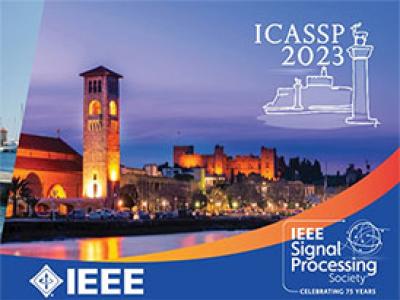
- Read more about MULTIMODAL EMOTION RECOGNITION BASED ON DEEP TEMPORAL FEATURES USING CROSS-MODAL TRANSFORMER AND SELF-ATTENTION
- Log in to post comments
Multimodal speech emotion recognition (MSER) is an emerging and challenging field of research due to its more robust characteristics than unimodal. However, in multimodal approaches, the interactive relations for model building using different modalities of speech representations for emotion recognition have not been well investigated yet. To address this issue, we introduce a new approach to capturing the deep temporal features of audio and text. The audio features are learned with a convolution neural network (CNN) and a Bi-directional Gated Recurrent Unit (Bi-GRU) network.
- Categories:
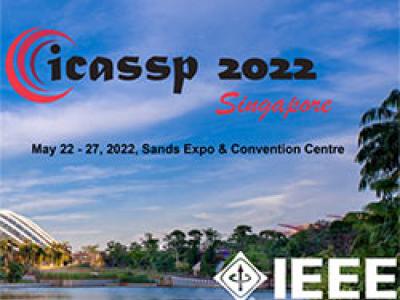
- Read more about ENABLING ON-DEVICE TRAINING OF SPEECH RECOGNITION MODELS WITH FEDERATED DROPOUT
- Log in to post comments
Federated learning can be used to train machine learning models on the edge on local data that never leave devices, providing privacy by default. This presents a challenge pertaining to the communication and computation costs associated with clients’ devices. These costs are strongly correlated with the size of the model being trained, and are significant for state-of-the-art automatic speech recognition models.We propose using federated dropout to reduce the size of client models while training a full-size model server-side.
- Categories:
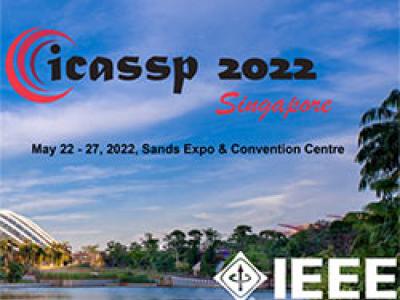
The recently proposed conformer architecture has been successfully used for end-to-end automatic speech recognition (ASR) architectures achieving state-of-the-art performance on different datasets. To our best knowledge, the impact of using conformer acoustic model for hybrid ASR is not investigated. In this paper, we present and evaluate a competitive conformer-based hybrid model training recipe. We study different training aspects and methods to improve worderror-rate as well as to increase training speed.
- Categories:
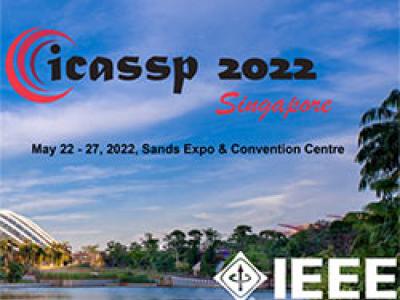
- Read more about poster of the paper 'End-to-End Speech Recognition from Federated Acoustic Models'
- 2 comments
- Log in to post comments
- Categories:
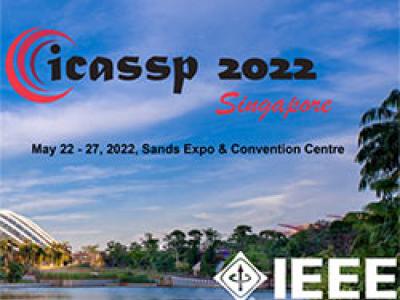
- Read more about slides for the paper 'End-to-End Speech Recognition from Federated Acoustic Models'
- 1 comment
- Log in to post comments
- Categories:
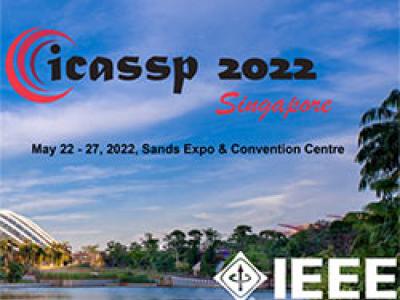
- Read more about Exploring Heterogeneous Characteristics of Layers in ASR Models for More Efficient Training
- Log in to post comments
Transformer-based architectures have been the subject of research aimed at understanding their overparameterization and the non-uniform importance of their layers. Applying these approaches to Automatic Speech Recognition, we demonstrate that the state-of-the-art Conformer models generally have multiple ambient layers. We study the stability of these layers across runs and model sizes, propose that group normalization may be used without disrupting their formation, and examine their correlation with model weight updates in each layer.
- Categories: