Documents
Poster
Source-Aware Context Network for Single-Channel Multi-speaker Speech Separation
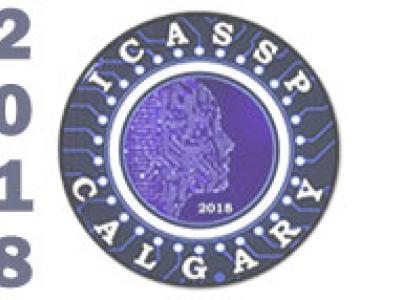
- Citation Author(s):
- Submitted by:
- Zengxi Li
- Last updated:
- 12 April 2018 - 9:42pm
- Document Type:
- Poster
- Document Year:
- 2018
- Event:
- Presenters:
- Zengxi Li
- Paper Code:
- 2474
- Categories:
- Log in to post comments
Deep learning based approaches have achieved promising performance in speaker-dependent single-channel multi-speaker speech separation.However, partly due to the label permutation problem, they may encounter difficulties in speaker-independent conditions. Recent methods address this problem by some assignment operations. Different from them, we propose a novel source-aware context network, which explicitly inputs speech sources as well as mixture signal. By exploiting the temporal dependency and continuity of the same source signal, the permutation order of outputs can be easily determined without any additional post-processing. Furthermore, a Multi-time-step Prediction Training strategy is proposed to address the mismatch between training and inference stages. Experimental results on benchmark WSJ0-2mix dataset revealed that our network achieved comparable or better results than state-of-the-art methods in both closed-set and open-set conditions, in terms of Signal-to-Distortion Ratio (SDR) improvement.
version2.pdf
