Documents
Poster
QUERY EXPANSION WITH DIFFUSION ON MUTUAL RANK GRAPHS
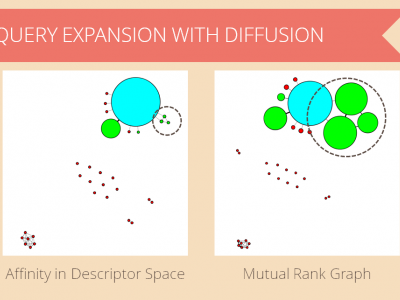
- Citation Author(s):
- Submitted by:
- Xiaomeng Wu
- Last updated:
- 12 April 2018 - 10:03pm
- Document Type:
- Poster
- Document Year:
- 2018
- Event:
- Presenters:
- Xiaomeng Wu
- Paper Code:
- 1054
- Categories:
- Log in to post comments
In query expansion for object retrieval, there is substantial danger of query drift, where irrelevant information is inferred from pseudo-relevant images to enrich the query. To address this issue, we propose a query expansion method from the viewpoint of diffusion. It explores the structure of highly ranked images in a topological space, assuming that false positives reside on different manifolds from the query. For this purpose, a mutual rank graph is defined on pseudo-relevant images, and their distribution is learned by diffusing their query similarities through the graph. The relevance of a database image can thus be obtained by marginalizing over the learned distribution. The mutual rank graph accounts for varying local density in the image space, leading to great robustness as regards query drift and high generalization ability. The proposed method experimentally shows a consistent boost in the performance of object retrieval with handcrafted features on standard benchmarks.
ICASSP18.2.pdf
