Documents
Presentation Slides
Bayesian anisotropic Gaussian model for audio source separation
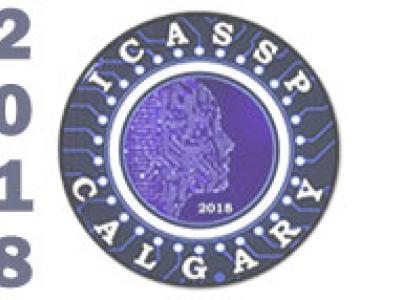
- Citation Author(s):
- Submitted by:
- Paul Magron
- Last updated:
- 16 June 2021 - 6:38am
- Document Type:
- Presentation Slides
- Document Year:
- 2018
- Event:
- Presenters:
- Paul Magron
- Categories:
- Log in to post comments
In audio source separation applications, it is common to model the sources as circular-symmetric Gaussian random variables, which is equivalent to assuming that the phase of each source is uniformly distributed. In this paper, we introduce an anisotropic Gaussian source model in which both the magnitude and phase parameters are modeled as random variables. In such a model, it becomes possible to promote a phase value that originates from a signal model and to adjust the relative importance of this underlying model-based phase constraint. We conduct Bayesian inference of the model through the derivation of an expectation-maximization algorithm for estimating the parameters. Experiments conducted on realistic music songs for a monaural source separation task, in an scenario where the variance parameters are assumed known, show that the proposed approach outperforms state-of-the-art techniques.