Documents
Poster
OPTIMAL POOLING OF COVARIANCE MATRIX ESTIMATES ACROSS MULTIPLE CLASSES
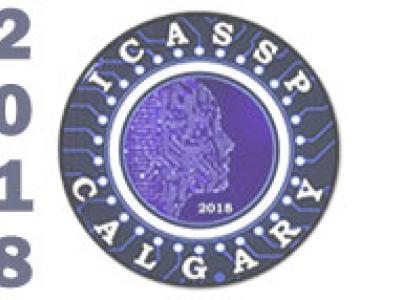
- Citation Author(s):
- Submitted by:
- Elias Raninen
- Last updated:
- 18 April 2018 - 11:40am
- Document Type:
- Poster
- Document Year:
- 2018
- Event:
- Presenters:
- Elias Raninen
- Paper Code:
- SPTM-P2.5
- Categories:
- Log in to post comments
The paper considers the problem of estimating the covariance matrices of multiple classes in a low sample support condition, where the data dimensionality is comparable to, or larger than, the sample sizes of the available data sets. In such conditions, a common approach is to shrink the class sample covariance matrices (SCMs) towards the pooled SCM. The success of this approach hinges upon the ability to choose the optimal regularization parameter. Typically, a common regularization level is shared among the classes and determined via a procedure based on cross-validation. We use class-specific regularization levels since this enables the derivation of the optimal regularization parameter for each class in terms of the minimum mean squared error (MMSE). The optimal parameters depend on the true unknown class population covariances. Consistent estimators of the parameters can, however, be easily constructed under the assumption that the class populations follow (unspecified) elliptically symmetric distributions. We demonstrate the performance of the proposed method via a simulation study as well as via an application to discriminant analysis using both synthetic and real data sets.