Documents
Poster
A Novel Learnable Dictionary Encoding Layer for End-to-End Language Identification
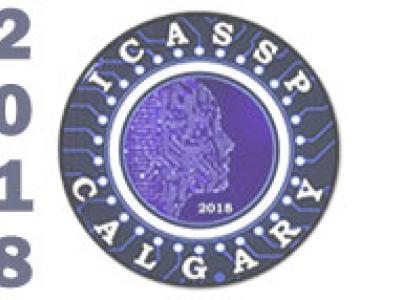
- Citation Author(s):
- Submitted by:
- Weicheng Cai
- Last updated:
- 13 April 2018 - 9:37am
- Document Type:
- Poster
- Document Year:
- 2018
- Event:
- Presenters:
- Weicheng Kai
- Paper Code:
- 3889
- Categories:
- Log in to post comments
A novel learnable dictionary encoding layer is proposed in this paper for end-to-end language identification. It is inline with the conventional GMM i-vector approach both theoretically and practically. We imitate the mechanism of traditional GMM training and Supervector encoding procedure on the top of CNN. The proposed layer can accumulate high-order statistics from variable-length input sequence and generate an utterance level fixed-dimensional vector representation. Unlike the conventional methods, our new approach provides an end-to-end learning framework, where the inherent dictionary are learned directly from the loss function. The dictionaries and the encoding representation for the classifier are learned jointly. The representation is orderless and therefore appropriate for language identification. We conducted a preliminary experiment on NIST LRE07 closed-set task, and the results reveal that our proposed dictionary encoding layer achieves significant error reduction comparing with the simple average pooling.