Documents
Poster
NEURAL ADAPTIVE IMAGE DENOISER
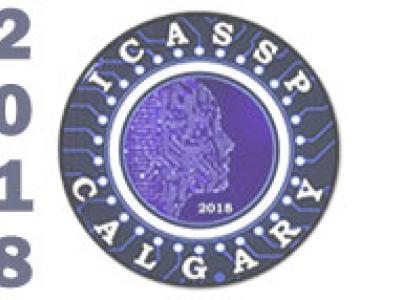
- Citation Author(s):
- Submitted by:
- Sungmin Cha
- Last updated:
- 14 April 2018 - 8:37am
- Document Type:
- Poster
- Document Year:
- 2018
- Event:
- Presenters:
- Sungmin Cha
- Paper Code:
- 3035
- Categories:
- Keywords:
- Log in to post comments
We propose a novel neural network-based adaptive image denoiser, dubbased as Neural AIDE. Unlike other neural network-based denoisers, which typically apply supervised training to learn a mapping from a noisy patch to a clean patch, we formulate to train a neural network to learn context- based affine mappings that get applied to each noisy pixel. Our formulation enables using SURE (Stein’s Unbiased Risk Estimator)-like estimated losses of those mappings as empirical risks to minimize. In results, we can combine both supervised training of the network parameters from a separate dataset and adaptive fine-tuning of them using the given noisy image subject to denoising. Our algorithm with a plain fully connected architecture is shown to attain a competitive denoising performance on benchmark datasets compared to the strong baselines. Furthermore, Neural AIDE can robustly correct the mismatched noise level in the supervised learning via fine-tuning, of which adaptivity is absent in other neural network-based denoisers.