Documents
Poster
Regularized SVD-based Video Frame Saliency for Unsupervised Activity Video Summarization
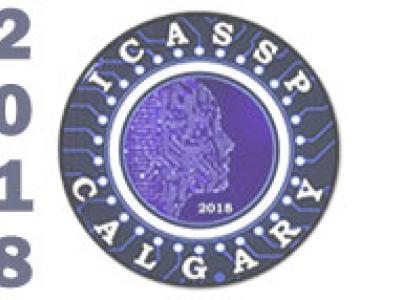
- Citation Author(s):
- Submitted by:
- Ioannis Mademlis
- Last updated:
- 18 April 2018 - 4:17am
- Document Type:
- Poster
- Document Year:
- 2018
- Event:
- Presenters:
- Ioannis Pitas
- Categories:
- Keywords:
- Log in to post comments
Storage, browsing and analysis of human activity videos can be significantly facilitated by automated video summarization. Unsupervised key-frame extraction remains the most widely applicable technique for summarizing activity videos. However, their specific properties make the problem difficult to solve. Typical relevant algorithms fall under the video frame clustering or the dictionary-of-representatives families, with salient dictionary learning having been recently proposed. Under this formulation, the video frames selected as key-frames are the ones which simultaneously best reconstruct the entire video and are salient compared to the rest. This paper improves upon such a method by replacing the video frame saliency estimation term with one based on Regularized SVD-based Low Rank Approximation, taking advantage of the well-established correlation between mid-range matrix singular values and salient regions. Extensive empirical evaluation showcases the high performance of both the salient dictionary learning framework and the specific proposed method.