Documents
Poster
Bayesian Sparse Signal Detection Exploiting Laplace Prior
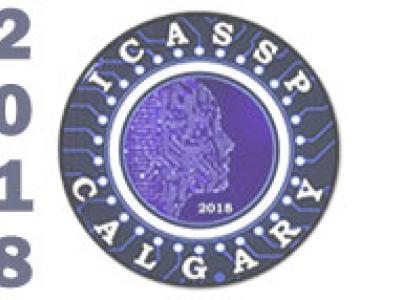
- Citation Author(s):
- Submitted by:
- Swatantra Kafle
- Last updated:
- 20 April 2018 - 1:55am
- Document Type:
- Poster
- Document Year:
- 2018
- Event:
- Presenters:
- Swatantra Kafle
- Paper Code:
- 4177
- Categories:
- Keywords:
- Log in to post comments
In this paper, we consider the problem of sparse signal detection with compressed measurements in a Bayesian framework. Multiple nodes in the network are assumed to observe sparse signals. Observations at each node are compressed via random projections and sent to a centralized fusion center. Motivated by the fact that reliable detection of the sparse signals does not require complete signal reconstruction, we propose two computationally efficient methods for constructing decision statistics for detection. First, using the Laplace prior directly to impose sparsity as widely considered in Bayesian Compressive Sensing (BCS), we develop an average likelihood ratio based detection method where the average is taken over the Laplace probability density function. Second, we exploit a three-stage hierarchical prior on the signal and construct decision statistics based on the noisy reconstruction (partial estimates) of the signals. Experimental results show that both average likelihood-based detection method and noisy-reconstruction based methods outperform most of the state-of-the-art algorithms.
ICASSP.pdf
