Documents
Poster
FULL-INFO TRAINING FOR DEEP SPEAKER FEATURE LEARNING
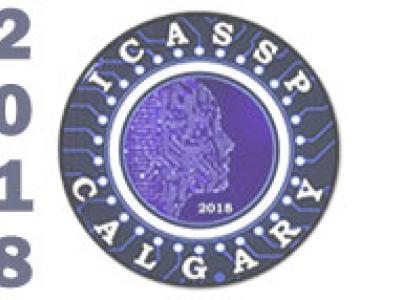
- Citation Author(s):
- Submitted by:
- Lantian Li
- Last updated:
- 20 April 2018 - 7:38am
- Document Type:
- Poster
- Document Year:
- 2018
- Event:
- Presenters:
- Miao Zhang
- Paper Code:
- 3967
- Categories:
- Log in to post comments
In recent studies, it has shown that speaker patterns can be learned from very short speech segments (e.g., 0.3 seconds) by a carefully designed convolutional & time-delay deep neural network (CT-DNN) model. By enforcing the model to discriminate the speakers in the training data, frame-level speaker features can be derived from the last hidden layer. In spite of its good performance, a potential problem of the present model is that it involves a parametric classifier, i.e., the last affine layer, which may consume some discriminative knowledge, thus leading to ‘information leak’ for the feature learning. This paper presents a full-info training approach that discards the parametric classifier and enforces all the discriminative knowledge learned by the feature net. Our experiments on the Fisher database demonstrate that this new training scheme can produce more coherent features, leading to consistent and notable performance improvement on the speaker verification task.