Documents
Poster
MULTICLASS WEIGHTED LOSS FOR INSTANCE SEGMENTATION OF CLUTTERED CELLS
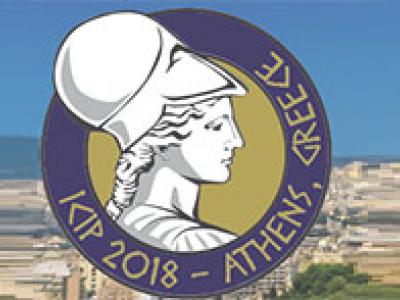
- Citation Author(s):
- Submitted by:
- Fidel Guerrero Pena
- Last updated:
- 5 October 2018 - 8:22am
- Document Type:
- Poster
- Document Year:
- 2018
- Event:
- Presenters:
- Alexandre Cunha
- Paper Code:
- 3186
- Categories:
- Log in to post comments
We propose a new multiclass weighted loss function for instance segmentation of cluttered cells. We are primarily motivated by the need of developmental biologists to quantify and model the behavior of blood T-cells which might help us in understanding their regulation mechanisms and ultimately help researchers in their quest for developing an effective immunotherapy cancer treatment. Segmenting individual touching cells in cluttered regions is challenging as the feature distribution on shared borders and cell foreground are similar thus difficulting discriminating pixels into proper classes. We present two novel weight maps applied to the weighted cross entropy loss function which take into account both class imbalance and cell geometry. Binary ground truth training data is augmented so the learning model can handle not only foreground and background but also a third touching class. This framework allows training using U-Net. Experiments with our formulations have shown superior results when compared to other similar schemes, outperforming binary class models with significant improvement of boundary adequacy and instance detection. We validate our results on manually annotated microscope images of T-cells.
postericip.pdf
