Documents
Presentation Slides
Defending DNN Adversarial Attacks with Pruning and Logits Augmentation
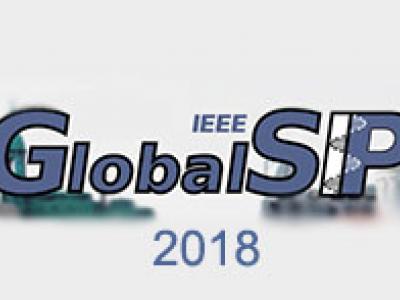
- Citation Author(s):
- Submitted by:
- Siyue Wang
- Last updated:
- 28 November 2018 - 8:40pm
- Document Type:
- Presentation Slides
- Document Year:
- 2018
- Event:
- Presenters:
- Pu Zhao
- Paper Code:
- 1228
- Categories:
- Log in to post comments
Deep neural networks (DNNs) have been shown to be powerful models and perform extremely well on many complicated artificial intelligent tasks. However, recent research found that these powerful models are vulnerable to adversarial attacks, i.e., intentionally added imperceptible perturbations to DNN inputs can easily mislead the DNNs with extremely high confidence. In this work, we enhance the robustness ofDNNs under adversarial attacks by using pruning method and logits augmentation, we achieve both effective defense against adversarial examples and DNN model compression. We have observed defense against adversarial attacks under the white box attack assumption. Our defense mechanisms work even better under the grey box attack assumption.