Documents
Presentation Slides
Gaussian process imputation of multiple financial series
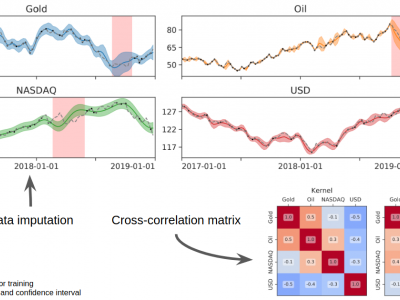
- Citation Author(s):
- Submitted by:
- Taco de Wolff
- Last updated:
- 13 May 2020 - 5:24pm
- Document Type:
- Presentation Slides
- Document Year:
- 2020
- Event:
- Presenters:
- Taco de Wolff
- Paper Code:
- SS-L2.3
- Categories:
- Log in to post comments
In Financial Signal Processing, multiple time series such as financial indicators, stock prices and exchange rates are strongly coupled due to their dependence on the latent state of the market and therefore they are required to be jointly analysed. We focus on learning the relationships among financial time series by modelling them through a multi-output Gaussian process (MOGP) with expressive covariance functions. Learning these market dependencies among financial series is crucial for the imputation and prediction of financial observations. The proposed model is validated experimentally on two real-world financial datasets for which their correlations across channels are analysed. We compare our model against other MOGPs and the independent Gaussian process on real financial data.