Documents
Presentation Slides
Monaural Speech Enhancement Using Intra-Spectral Recurrent Layers In The Magnitude And Phase Responses
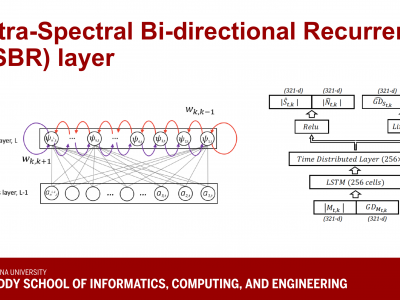
- Citation Author(s):
- Submitted by:
- KHANDOKAR MD NAYEM
- Last updated:
- 15 May 2020 - 2:02am
- Document Type:
- Presentation Slides
- Document Year:
- 2020
- Event:
- Presenters:
- Khandokar Md. Nayem
- Paper Code:
- SPE-L6.4
- Categories:
- Log in to post comments
Speech enhancement has greatly benefited from deep learning. Currently, the best performing deep architectures use long short-term memory (LSTM) recurrent neural networks (RNNs) to model short and long temporal dependencies. These approaches, however, underutilize or ignore spectral-level dependencies within the magnitude and phase responses, respectively. In this paper, we propose a deep learning architecture that leverages both temporal and spectral dependencies within the magnitude and phase responses. More specifically, we first train a LSTM network to predict both the spectral-magnitude response and group delay, where this model captures temporal correlations. We then introduce Markovian recurrent connections in the output layers to capture spectral dependencies within the magnitude and phase responses. We compare our approach with traditional enhancement approaches and approaches that consider spectral dependencies within a single time frame. The results show that considering the within-frame spectral dependencies leads to improvements.