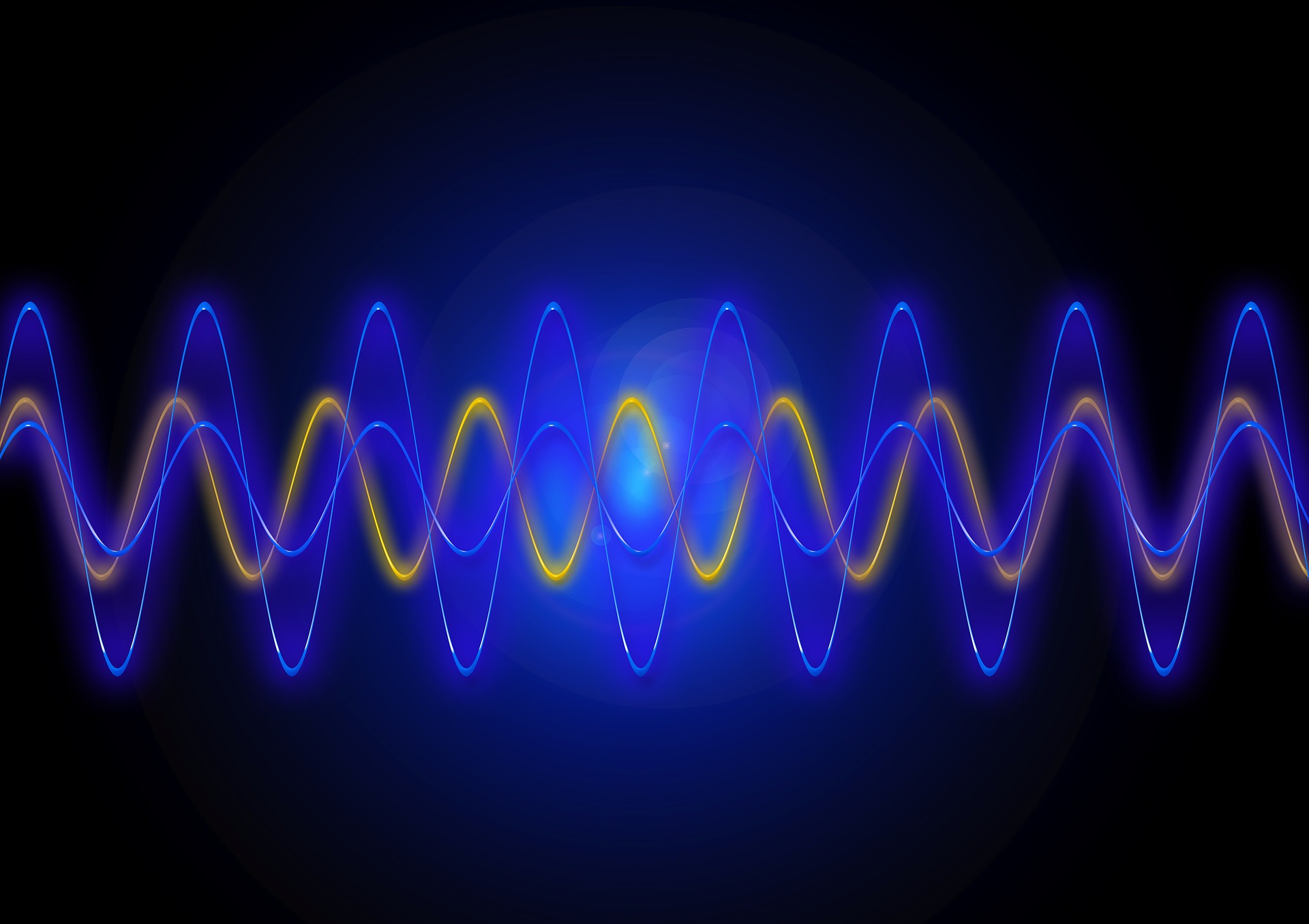
- Read more about AUDIO-VISUAL ACTIVE SPEAKER EXTRACTION FOR SPARSELY OVERLAPPED MULTI-TALKER SPEECH
- Log in to post comments
Target speaker extraction aims to extract the speech of a specific speaker from a multi-talker mixture as specified by an auxiliary reference. Most studies focus on the scenario where the target speech is highly overlapped with the interfering speech. However, this scenario only accounts for a small percentage of real-world conversations. In this paper, we aim at the sparsely overlapped scenarios in which the auxiliary reference needs to perform two tasks simultaneously: detect the activity of the target speaker and disentangle the active speech from any interfering speech.
- Categories:
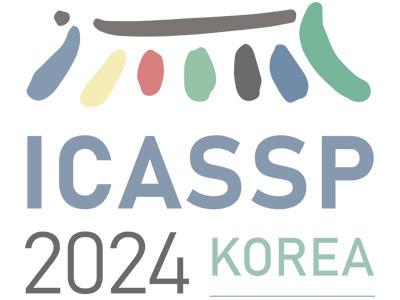
Advancements in generative algorithms promise new heights in what can be achieved, for example, in the speech enhancement domain. Beyond the ubiquitous noise reduction, destroyed speech components can now be restored—something not previously achievable. These emerging advancements create both opportunities and risks, as speech intelligibility can be impacted in a multitude of beneficial and detrimental ways. As such, there exists a need for methods, materials and tools for enabling rapid and effective assessment of speech intelligibility.
- Categories:
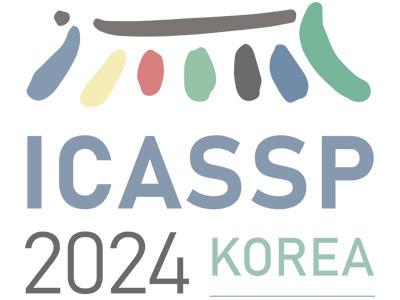
- Read more about Boosting Speech Enhancement with Clean Self-Supervised Features Via Conditional Variational Autoencoders
- Log in to post comments
Recently, Self-Supervised Features (SSF) trained on extensive speech datasets have shown significant performance gains across various speech processing tasks. Nevertheless, their effectiveness in Speech Enhancement (SE) systems is often suboptimal due to insufficient optimization for noisy environments. To address this issue, we present a novel methodology that directly utilizes SSFs extracted from clean speech for enhancing SE models. Specifically, we leverage the clean SSFs for latent space modeling within the Conditional Variational Autoencoder (CVAE) framework.
- Categories:
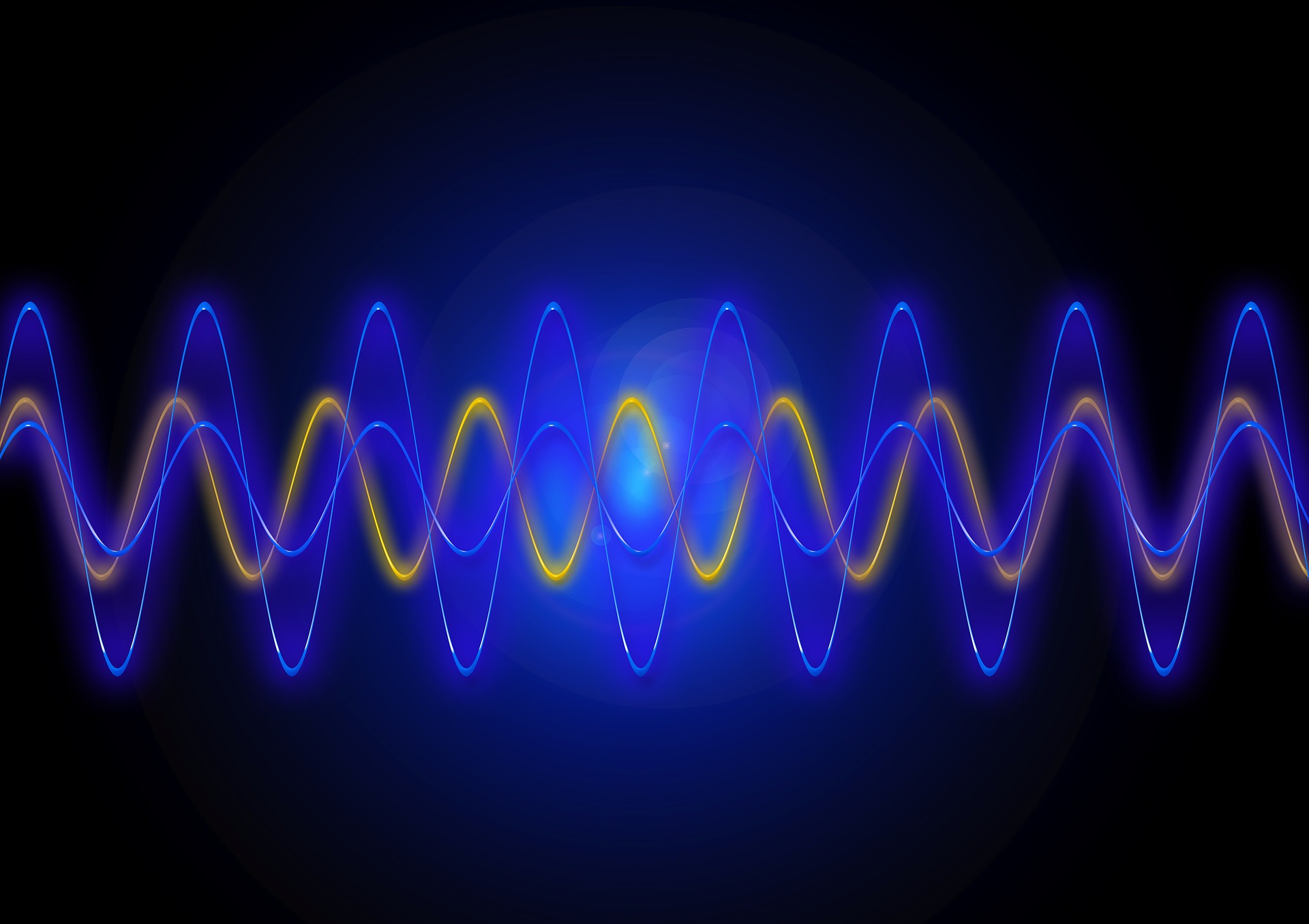
- Read more about Boosting Speech Enhancement with Clean Self-Supervised Features Via Conditional Variational Autoencoders
- Log in to post comments
Recently, Self-Supervised Features (SSF) trained on extensive speech datasets have shown significant performance gains across various speech processing tasks. Nevertheless, their effectiveness in Speech Enhancement (SE) systems is often suboptimal due to insufficient optimization for noisy environments. To address this issue, we present a novel methodology that directly utilizes SSFs extracted from clean speech for enhancing SE models. Specifically, we leverage the clean SSFs for latent space modeling within the Conditional Variational Autoencoder (CVAE) framework.
- Categories:
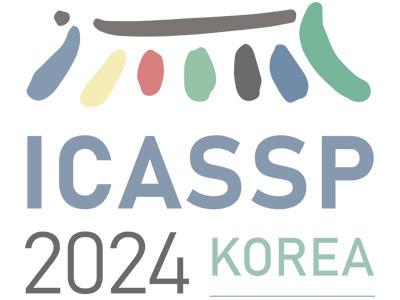
- Read more about slides for av2wav
- 1 comment
- Log in to post comments
Speech enhancement systems are typically trained using pairs of clean and noisy speech. In audio-visual speech enhancement
- Categories:
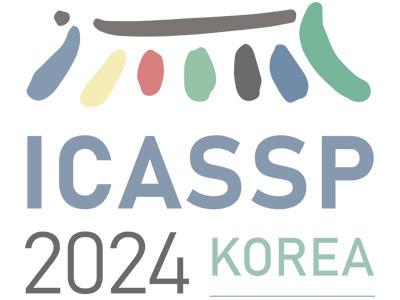
- Read more about DIFFUSION-BASED SPEECH ENHANCEMENT IN MATCHED AND MISMATCHED CONDITIONS USING A HEUN-BASED SAMPLER
- Log in to post comments
Diffusion models are a new class of generative models that have recently been applied to speech enhancement successfully. Previous works have demonstrated their superior performance in mismatched conditions compared to state-of-the art discriminative models. However, this was investigated with a single database for training and another one for testing, which makes the results highly dependent on the particular databases. Moreover, recent developments from the image generation literature remain largely unexplored for speech enhancement.
- Categories:
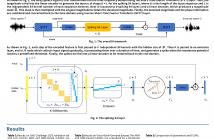
- Read more about SPIKING STRUCTURED STATE SPACE MODEL FOR MONAURAL SPEECH ENHANCEMENT
- Log in to post comments
Speech enhancement seeks to extract clean speech from noisy signals. Traditional deep learning methods face two challenges: efficiently using information in long speech sequences and high computational costs. To address these, we introduce the Spiking Structured State Space Model (Spiking-S4). This approach merges the energy efficiency of Spiking Neural Networks (SNN) with the long-range sequence modeling capabilities of Structured State Space Models (S4), offering a compelling solution.
- Categories:
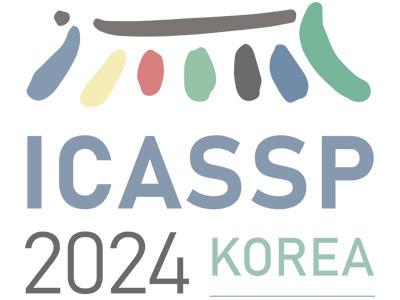
- Read more about MossFormer2: Combining Transformer and RNN-Free Recurrent Network for Enhanced Time-Domain Monaural Speech Separation
- 1 comment
- Log in to post comments
Our previously proposed MossFormer has achieved promising performance in monaural speech separation. However, it predominantly adopts a self-attention-based MossFormer module, which tends to emphasize longer-range, coarser-scale dependencies, with a deficiency in effectively modelling finer-scale recurrent patterns. In this paper, we introduce a novel hybrid model that provides the capabilities to model both long-range, coarse-scale dependencies and fine-scale recurrent patterns by integrating a recurrent module into the MossFormer framework.
- Categories:
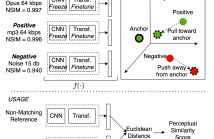
- Read more about NOMAD: Non-Matching Audio Distance
- Log in to post comments
This paper presents NOMAD (Non-Matching Audio Distance), a differentiable perceptual similarity metric that measures the distance of a degraded signal against non-matching references. The proposed method is based on learning deep feature embeddings via a triplet loss guided by the Neurogram Similarity Index Measure (NSIM) to capture degradation intensity. During inference, the similarity score between any two audio samples is computed through Euclidean distance of their embeddings. NOMAD is fully unsupervised and can be used in general perceptual audio tasks for audio analysis e.g.
- Categories:
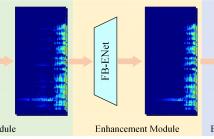
- Read more about RENet: A Time-Frequency Domain General Speech Restoration Network for ICASSP 2024 Speech Signal Improvement Challenge
- Log in to post comments
The ICASSP 2024 Speech Signal Improvement (SSI) Challenge seeks to address speech quality degradation problems in telecommunication systems. In this context, this paper proposes RENet, a time-frequency (T-F) domain method leveraging complex spectrum mapping to mitigate speech distortions. Specifically, the proposed RENet is a multi-stage network. First, TF-GridGAN was designed to recover the degraded speech with a generative adversarial network (GAN). Second, a full-band enhancement module was introduced to eliminate residual noises and artifacts existed in the output of TF-GridGAN.
2024.4.19.pptx

- Categories: