Documents
Research Manuscript
ICIP 2020 Paper #2783: FEW SHOT LEARNING FOR POINT CLOUD DATA USING MODEL AGNOSTIC META LEARNING
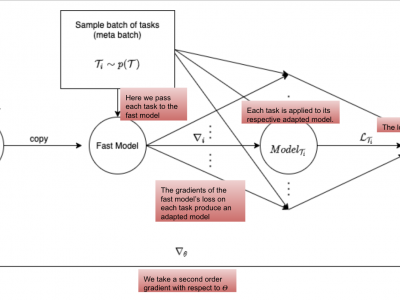
- Citation Author(s):
- Submitted by:
- Rishi Puri
- Last updated:
- 4 November 2020 - 10:59am
- Document Type:
- Research Manuscript
- Document Year:
- 2020
- Event:
- Presenters:
- Rishi Puri
- Categories:
- Log in to post comments
The ability of deep neural networks to extract complex statistics and learn high level features from vast datasets is proven.Yet current deep learning approaches suffer from poor sample efficiency in stark contrast to human perception. Fewshot learning algorithms such as matching networks or ModelAgnostic Meta Learning (MAML) mitigate this problem, enabling fast learning with few examples. In this paper, we ex-tend the MAML algorithm to point cloud data using a Point-Net Architecture. We constructN×K-shot classification tasks from the ModelNet40 point cloud dataset to show that this method performs classification as well as supervised deep learning methods with the added benefit of being able to adapt after a single gradient step on a singleN×K task. We empirically search for optimal values of N and K for few shot classification and show our method to achieve 90% meta test accuracy compared to traditional PointNet with 89.2%. We also adapt a meta-trained PointNet to a support set of 9,N=3,K= 3, never before seen point clouds which are drawn from an entirely different dataset, ShapeNet. Once adapted the model achieves 7.1/9 classification accuracy on average across 100 query sets of the same classes with new, unique instances. This result far exceeds the supervised StochasticGradient Descent (SGD) training result of 3.1/9 accuracy on the query sets which is equivalent to a random baseline.