Documents
Presentation Slides
Attribute-Decomposable Motion Compression Network for 3D MoCap Data
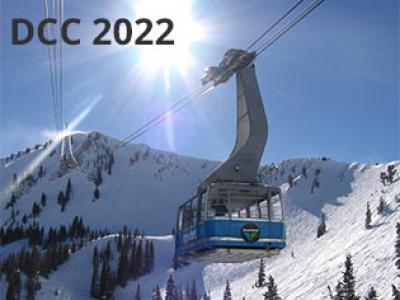
- Citation Author(s):
- Submitted by:
- Ju Dai
- Last updated:
- 25 February 2022 - 7:51am
- Document Type:
- Presentation Slides
- Document Year:
- 2022
- Event:
- Presenters:
- Ju Dai
- Paper Code:
- DCC-103
- Categories:
- Log in to post comments
Motion Capture (MoCap) data is one type of fundamental asset for the digital entertainment. The progressively increasing 3D applications make MoCap data compression unprecedentedly important. In this paper, we propose an end-to-end attribute-decomposable motion compression network using the AutoEncoder architecture. Specifically, the algorithm consists of an LSTM-based encoder-decoder for compression and decompression. The encoder module decomposes human motion into multiple uncorrelated semantic attributes, including action content, arm space, and motion mirror. The decoder module is responsible for reconstructing vivid motion based on the decomposed high-level characteristics. Our method is computationally efficient with powerful compression ability, outperforming the state-of-the-art methods in terms of compression rate and compression error. Furthermore, our model can generate new motion data given a combination of different motion attributes while existing methods have no such capability.