Documents
Poster
Comparison and extension of autoencoder models for uni- and multivariate signal compression in IIoT
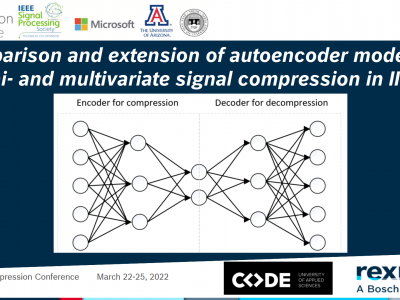
- Citation Author(s):
- Submitted by:
- Julia Rosenberger
- Last updated:
- 3 March 2022 - 4:52am
- Document Type:
- Poster
- Document Year:
- 2022
- Event:
- Presenters:
- Julia Rosenberger
- Paper Code:
- Paper ID 133
- Categories:
- Log in to post comments
In the production plants in the era of industry 4.0, every unit in the production process will generate and transmit data. Transmitting this data in real-time is not unrestrictedly feasible due to the limited bandwidth of the Internet, and processing on the edge device of the unit is also not conceivable due to the limited computing capacity of the device. For solving these constraints, a comparison of two generalizing neural networks with an autoencoder (AE) structure, more precisely one based on convolutional variational AE with Restricted Boltzmann Machines (RBMs) and the other one on a recurrent AE, is drawn. The two approaches are adapted to the use case of streaming data compression in the industrial Internet of Things (IIoT) and compared on two publicly available data sets. Both models are further extended to the capability of compressing uni- as well as multivariate data.