Documents
Presentation Slides
SEISMIC FAULT IDENTIFICATION USING GRAPH HIGH-FREQUENCY COMPONENTS AS INPUT TO GRAPH CONVOLUTIONAL NETWORK
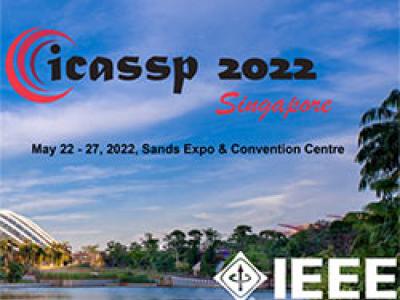
- Citation Author(s):
- Submitted by:
- Patitapaban Palo
- Last updated:
- 7 May 2022 - 7:25am
- Document Type:
- Presentation Slides
- Document Year:
- 2022
- Event:
- Presenters:
- Patitapaban Palo
- Paper Code:
- 4667
- Categories:
- Log in to post comments
Many activities such as drilling and exploration in the oil and gas industries rely on identifying seismic faults. Using graph high-frequency components as inputs to a graph convolutional network, we propose a method for detecting faults in seismic data. In Graph Signal Processing (GSP), digital signal processing (DSP) concepts are mapped to define the processing techniques for signals on graphs. As a first step, we extract patches of the seismic data centered around the points of concern. Each patch is then represented in a graph domain, with the seismic amplitudes as the graph signals. We attenuate the low-frequency components of the signal with the aid of a graph high-pass filter. By applying the graph Fourier transform, we obtain the graph high-frequency components. These graph high-frequency components act as inputs to a graph convolutional network (GCN). By classifying the patches using GCN, we identify the faults in data.