Documents
Research Manuscript
PSEUDO-LABEL BASED SUPERVISED CONTRASTIVE LOSS FOR ROBUST SPEECH REPRESENTATIONS
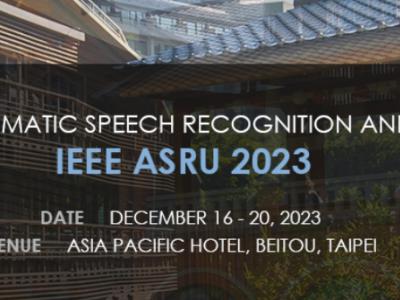
- DOI:
- 10.60864/53g4-7z10
- Citation Author(s):
- Submitted by:
- Sriram Ganapathy
- Last updated:
- 15 December 2023 - 12:10pm
- Document Type:
- Research Manuscript
- Document Year:
- 2023
- Presenters:
- Varun Krishna
- Categories:
- Log in to post comments
The self supervised learning (SSL) of speech, with discrete tokenization (pseudo-labels), while illustrating performance improvements in low-resource speech recognition, has faced challenges in achieving context invariant and noise robust representations. In this paper,we propose a self-supervised framework based on contrastive loss of the pseudo-labels, obtained from an offline k-means quantizer (tokenizer). We refer to the proposed setting as pseudo-con. The pseudocon loss, within a batch of training, allows the model to cluster theinstances of the same pseudo-label while separating the instances of a different pseudo-label. The proposed pseudo-con loss can also be combined with the cross entropy loss, commonly used in selfsupervised learning schemes. We demonstrate the effectiveness ofthe pseudo-con loss applied for various SSL techniques, like hidden unit bidirectional encoder representations from transformers (HuBERT), best random quantizer (BEST-RQ) and hidden unit clustering (HUC).
Comments
Published at IEEE ASRU
Published at IEEE ASRU Workshop 2023.