Documents
Poster
Small-Footprint Convolutional Neural Network with reduced feature map for Voice Activity Detection
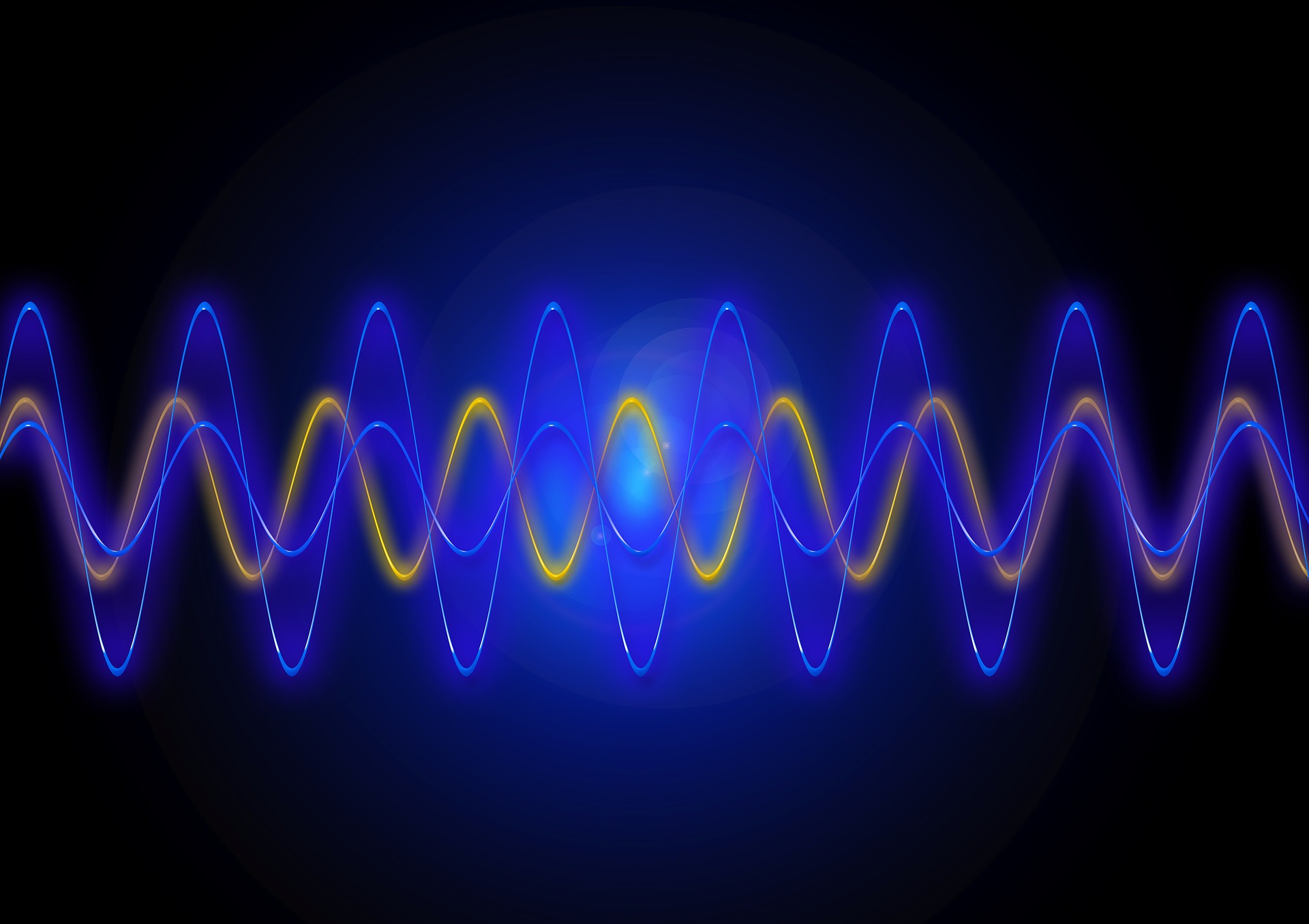
- Citation Author(s):
- Submitted by:
- Hwabyeong Chae
- Last updated:
- 4 April 2024 - 3:16am
- Document Type:
- Poster
- Categories:
- Log in to post comments
By using Voice Activity Detection (VAD) as a preprocessing step, hardware-efficient implementations are possible for speech applications that need to run continuously in severely resource-constrained environments. For this purpose, we propose TinyVAD, which is a new convolutional neural network(CNN) model that executes extremely efficiently with a small memory footprint. TinyVAD uses an input pixel matrix partitioning method, termed patchify, to downscale the resolution of the input spectrogram. The hidden layers use a sequence of special convolutional structures with bypass links, referred to as CSPTiny layers. The proposed model is evaluated and compared with previous VAD methods using a diverse set of noisy environmental datasets. TinyVAD executes 3.13 times faster, utilizes only 12.5% as many multiplications, and requires only 13.0% as many parameters when compared to the previous state-of-the-art.