Documents
Poster
META-KNOWLEDGE ENHANCED DATA AUGMENTATION FOR FEDERATED PERSON RE-IDENTIFICATION
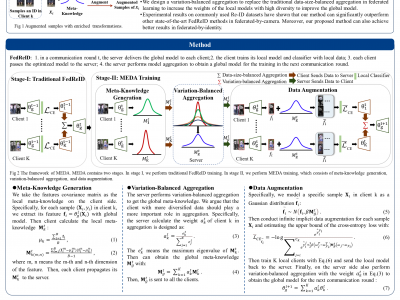
- Citation Author(s):
- Submitted by:
- Chunli Song
- Last updated:
- 8 April 2024 - 3:07am
- Document Type:
- Poster
- Paper Code:
- SPCOM-P1.7
- Categories:
- Log in to post comments
Recently, federated learning has been introduced into person re-identification (Re-ID) to avoid personal image leakage in traditional centralized training. To address the key issue of statistic heterogeneity in different clients, several optimization methods have been proposed to alleviate the bias of the local models. However, besides statistic heterogeneity, feature heterogeneity (e.g., various angles, different illuminations) in different clients is more challenging in federated Re-ID. In this paper, we propose a meta-knowledge enhanced data augmentation method, where the global cross semantic feature transformations are provided to each client to perform local infinite augmentation to reduce the feature difference in different clients. Specifically, to capture the cross semantic feature transformations in each client, we calculate the covariance matrix of features with the local dataset as the transferable meta-knowledge. Then, this local meta-knowledge is propagated to the server for global aggregation. Subsequently, the aggregated meta-knowledge is sent back to each client for infinite data augmentation. Moreover, since the covariance matrix indicates variations in a client, we design a variation-balanced aggregation to replace the traditional data-size-balanced aggregation. To imitate the more challenging scenario of feature heterogeneity, we focus on the federated-by-camera setting to conduct experiments, where images collected in a camera are regarded as the dataset of a client. Extensive experimental results show that our method outperforms other state-of-the-art methods. Code is available at https://github.com/songchunli1999/MEDA.