Documents
Presentation Slides
ESVC: Combining Adaptive Style Fusion and Multi-Level Feature Disentanglement for Expressive Singing Voice Conversion
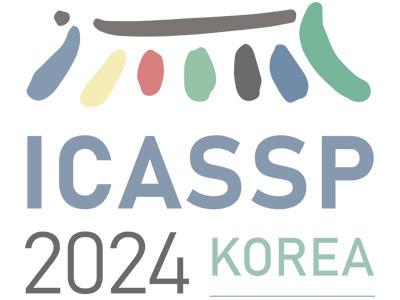
- DOI:
- 10.60864/bsek-cw36
- Citation Author(s):
- Submitted by:
- Minchuan Chen
- Last updated:
- 6 June 2024 - 10:54am
- Document Type:
- Presentation Slides
- Document Year:
- 2024
- Event:
- Presenters:
- Minchuan Chen
- Paper Code:
- 8178
- Categories:
- Log in to post comments
Nowadays, singing voice conversion (SVC) has made great strides in both naturalness and similarity for common SVC with a neutral expression. However, besides singer identity, emotional expression is also essential to convey the singer's emotions and attitudes, but current SVC systems can not effectively support it. In this paper, we propose an expressive SVC framework called ESVC, which can convert singer identity and emotional style simultaneously. ESVC combines the ideas of style fusion and feature disentanglement, seeking to maximize fidelity in terms of emotional style and singer identity. Firstly, for style information penetration, we employ adaptive instance normalization (AdaIN) to fuse the content feature and style feature. Secondly, given the possibility of information leakage, two disentanglement-oriented methods are introduced to decouple different kinds of singing features. Mutual information (MI) is used to reduce the correlation between linguistic content, fundamental frequency (F0) and expressive feature, while adversarial triplet loss is exerted for decoupling identity and emotional elements. To the best of our knowledge, ESVC is the first SVC system to jointly convert singer identity and emotional style. Objective and subjective experiments demonstrate that our system significantly outperforms the state-of-the-art SVC model in terms of style expressiveness.