Documents
Presentation Slides
DCL-NET DUAL CONTRASTIVE LEARNING NETWORK FOR SEMI-SUPERVISED MULTI-ORGAN SEGMENTATION
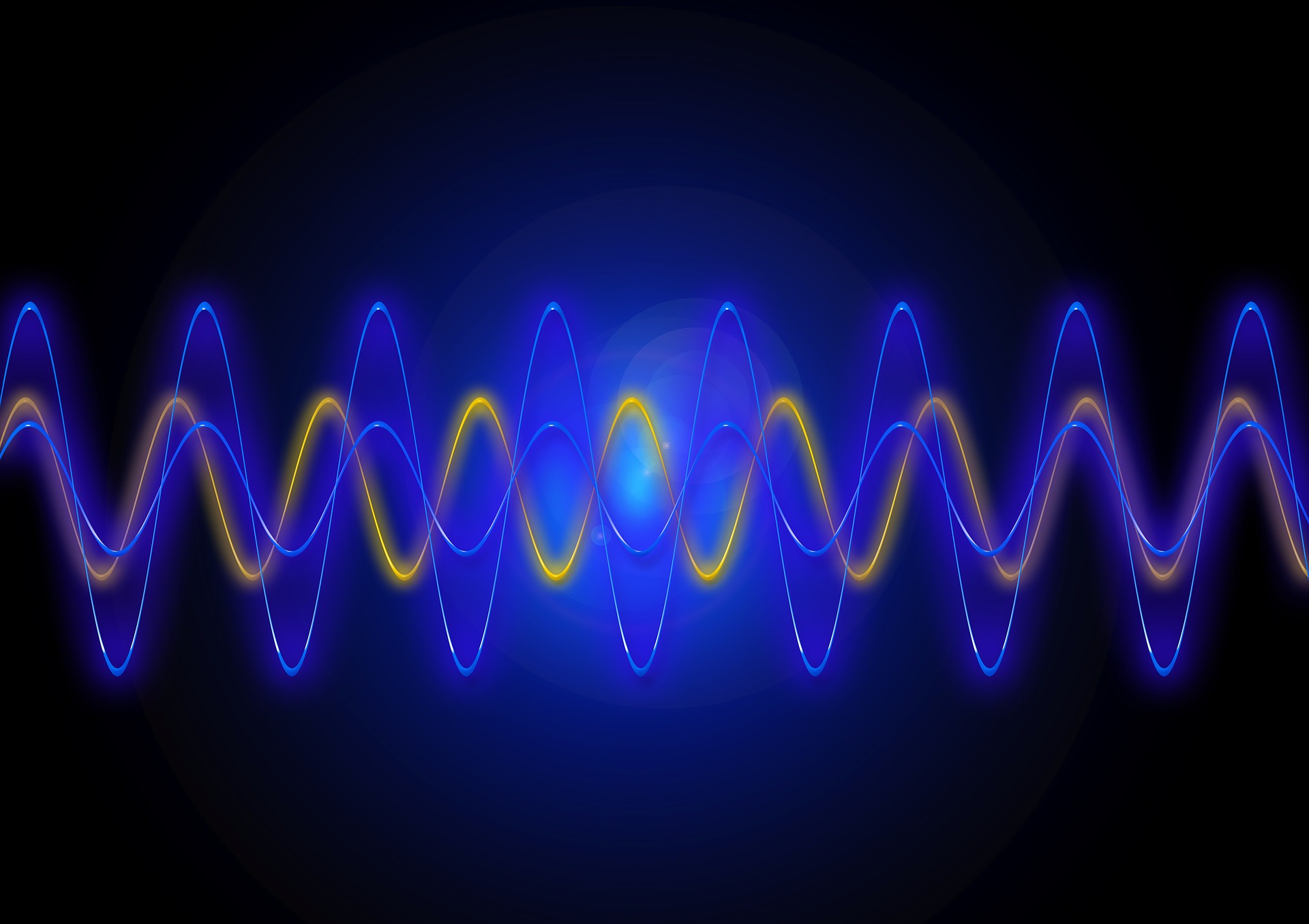
- DOI:
- 10.60864/eppj-w705
- Citation Author(s):
- Submitted by:
- Lu Wen
- Last updated:
- 6 June 2024 - 10:28am
- Document Type:
- Presentation Slides
- Document Year:
- 2024
- Presenters:
- Lu Wen
- Paper Code:
- BISP-L2.4
- Categories:
- Log in to post comments
Semi-supervised learning (SSL) is a sound measure to relieve the strict demand of abundant annotated datasets, especially for challenging multi-organ segmentation (MoS). However, most existing SSL methods predict pixels in a single image independently, ignoring the relations among images and categories. In this paper, we propose a two-stage Dual Contrastive Learning Network (DCL-Net) for semi-supervised MoS, which utilizes global and local contrastive learning to strengthen the relations among images and classes. Concretely, in Stage Ⅰ, we develop a similarity-guided global contrastive learning to explore the implicit continuity and similarity among images and learn global context. Then, in Stage Ⅱ, we present an organ-aware local contrastive learning to further attract the class representations. To ease the computation burden, we introduce a mask center computation algorithm to compress the category representations for local contrastive learning. Experiments conducted on the public 2017 ACDC dataset and an in-house RC-OARs dataset has demonstrated the superior performance of our method.