Documents
Presentation Slides
THE USTC-NERCSLIP SYSTEMS FOR THE ICMC-ASR CHALLENGE
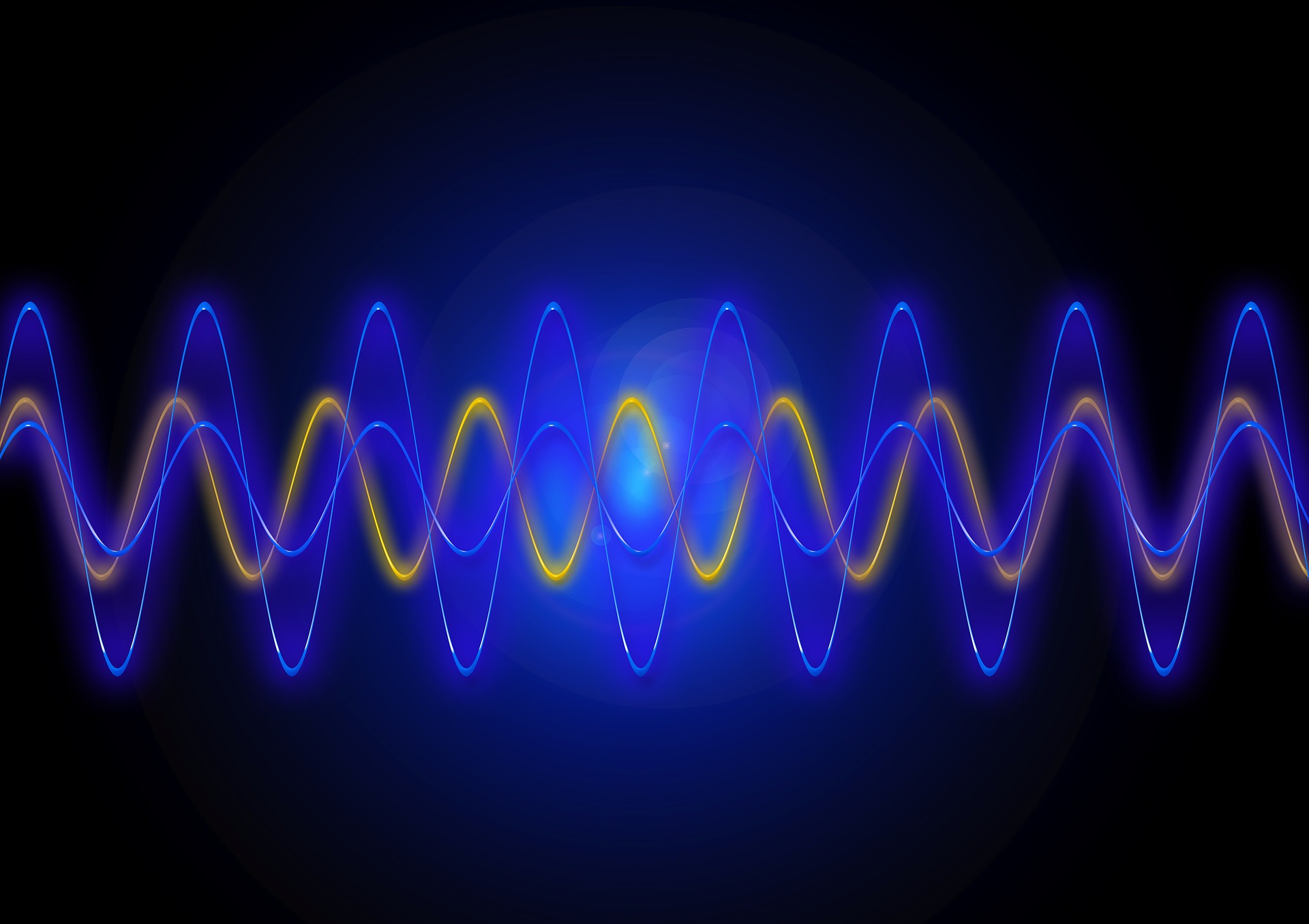
- DOI:
- 10.60864/c72c-zq13
- Citation Author(s):
- Submitted by:
- YICHI WANG
- Last updated:
- 6 June 2024 - 10:28am
- Document Type:
- Presentation Slides
- Categories:
- Log in to post comments
This report describes the submitted system to the In-Car Multi-Channel Automatic Speech Recognition (ICMC-ASR) challenge, which considers the ASR task with multi-speaker overlapping and Mandarin accent dynamics in the ICMC case. We implement the front-end speaker diarization using the self-supervised learning representation based multi-speaker embedding and beamforming using the speaker position, respectively. For ASR, we employ an iterative pseudo-label generation method based on fusion model to obtain text labels of unsupervised data. To mitigate the impact of accent, an Accent-ASR framework is proposed, which captures pronunciation-related accent features at a fine-grained level and linguistic information at a coarse-grained level. On the ICMC-ASR eval set, the proposed system achieves a CER of 13.16% on track 1 and a cpCER of 21.48% on track 2, which significantly outperforms the official baseline system and obtains the first rank on both tracks.