Documents
Presentation Slides
MMS: Morphology-mixup Stylized Data Generation for Single Domain Generalization in Medical Image Segmentation
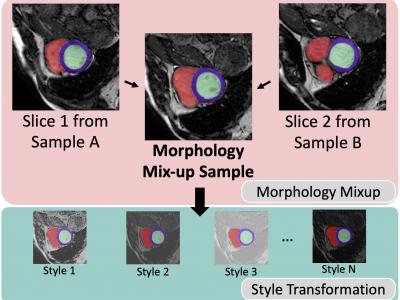
- DOI:
- 10.60864/ea7t-4925
- Citation Author(s):
- Submitted by:
- xiaochen he
- Last updated:
- 6 June 2024 - 10:28am
- Document Type:
- Presentation Slides
- Document Year:
- 2024
- Event:
- Presenters:
- Xiaochen He
- Paper Code:
- BISP-L2.6
- Categories:
- Log in to post comments
Single-source domain generalization in medical image segmentation is a challenging yet practical task, as domain shift commonly exists across medical datasets.
Previous works have attempted to alleviate this problem through adversarial data augmentation or random-style transformation.
However, these approaches neither fully leverage medical information nor consider the morphological structure alterations.
To address these limitations and enhance the fidelity and diversity of the augmented data,
we propose a Morphology-Mixup Stylized data generation (MMS) method,
which expands source data from a new morphological perspective, guided by the characteristics of medical imaging.
Specifically, we design a Mixed Dual-stream Auto-Encoder (MDs-AE) to simulate the morphology changes between medical image slices and mix the morphology of two slices.
In addition, we introduce a feature consistency strategy to improve the effectiveness of morphology mixing.
The trained MDs-AE with a random styler is used to generate data that vary in both morphology and style to enhance the generalization ability of the segmentation network.
Extensive experimental results demonstrate that MMS is effective and outperforms the state-of-the-art on three cross-domain segmentation tasks.