Documents
Presentation Slides
MTIDNET: A MULTIMODAL TEMPORAL INTEREST DETECTION NETWORK FOR VIDEO SUMMARIZATION
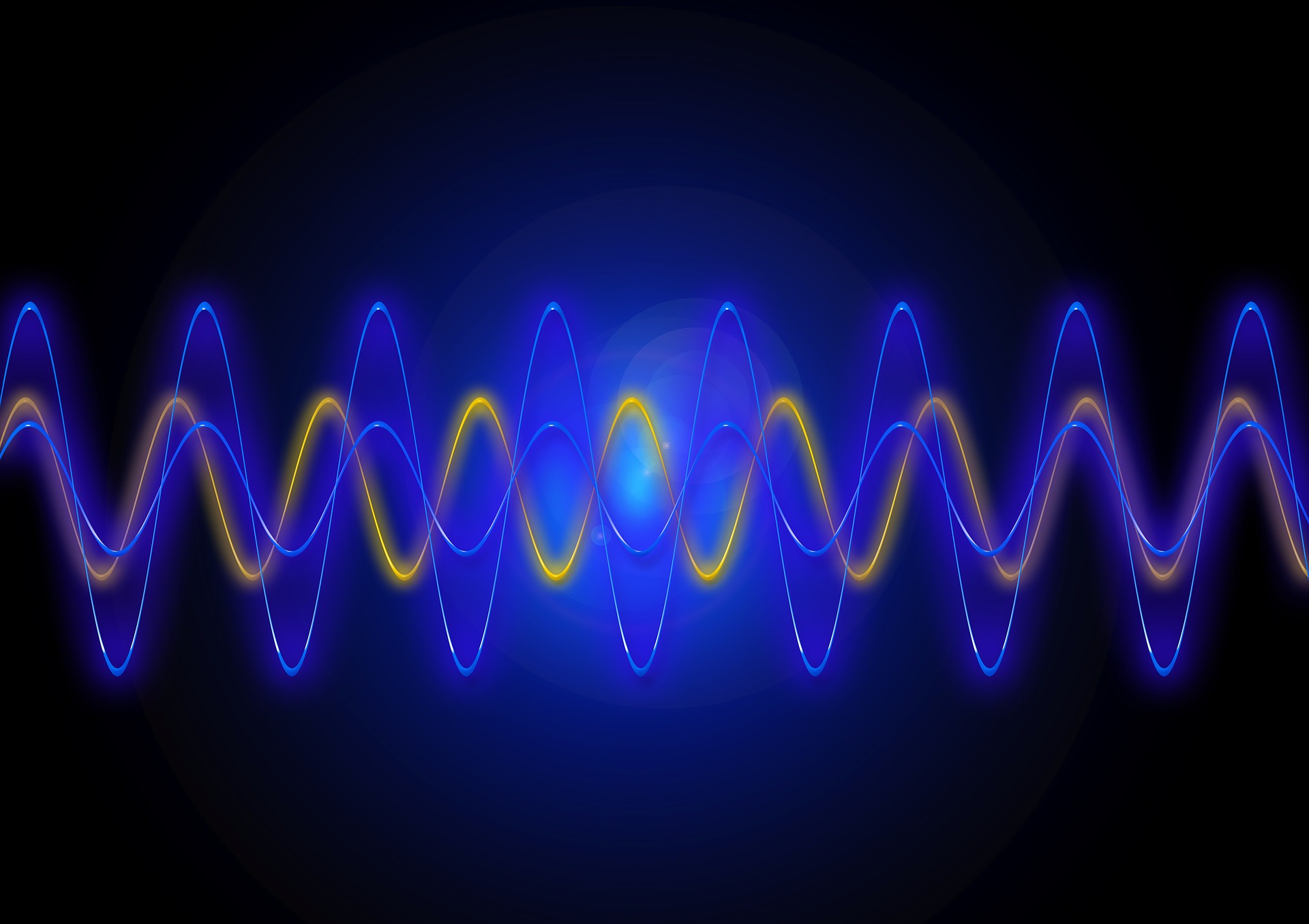
- DOI:
- 10.60864/qy8k-kz80
- Citation Author(s):
- Submitted by:
- Xiaoyan Tian
- Last updated:
- 6 June 2024 - 10:27am
- Document Type:
- Presentation Slides
- Categories:
- Log in to post comments
Video summarization involves creating a succinct overview by merging the valuable parts of a video. Existing video summarization
methods approach this task as a problem of selecting keyframes
by frame- and shot-level techniques with unimodal or bimodal information. Besides underestimated inter-relations between various
configurations of modality embedding spaces, current methods are
also limited in their ability to maintain the integrity of the semantics within the same summary segment. To address these issues,
we propose a novel multimodal temporal interest detection network
(MTIDNet), to learn multimodal features in the fine- and coarsegrained embedding spaces using the mutual cross fusion layer. Furthermore, we design a temporal interest detection network to predict
the importance scores and boundaries of each temporal segment that
possesses local and global features across shots. Experimental results demonstrate the effectiveness of our MTIDNet on challenging
datasets (SumMe and TVSum).
icassp.pptx
