Documents
Supplementary material
Supplementary Material for Effective relationship between characteristics of training data and learning progress on knowledge distillation
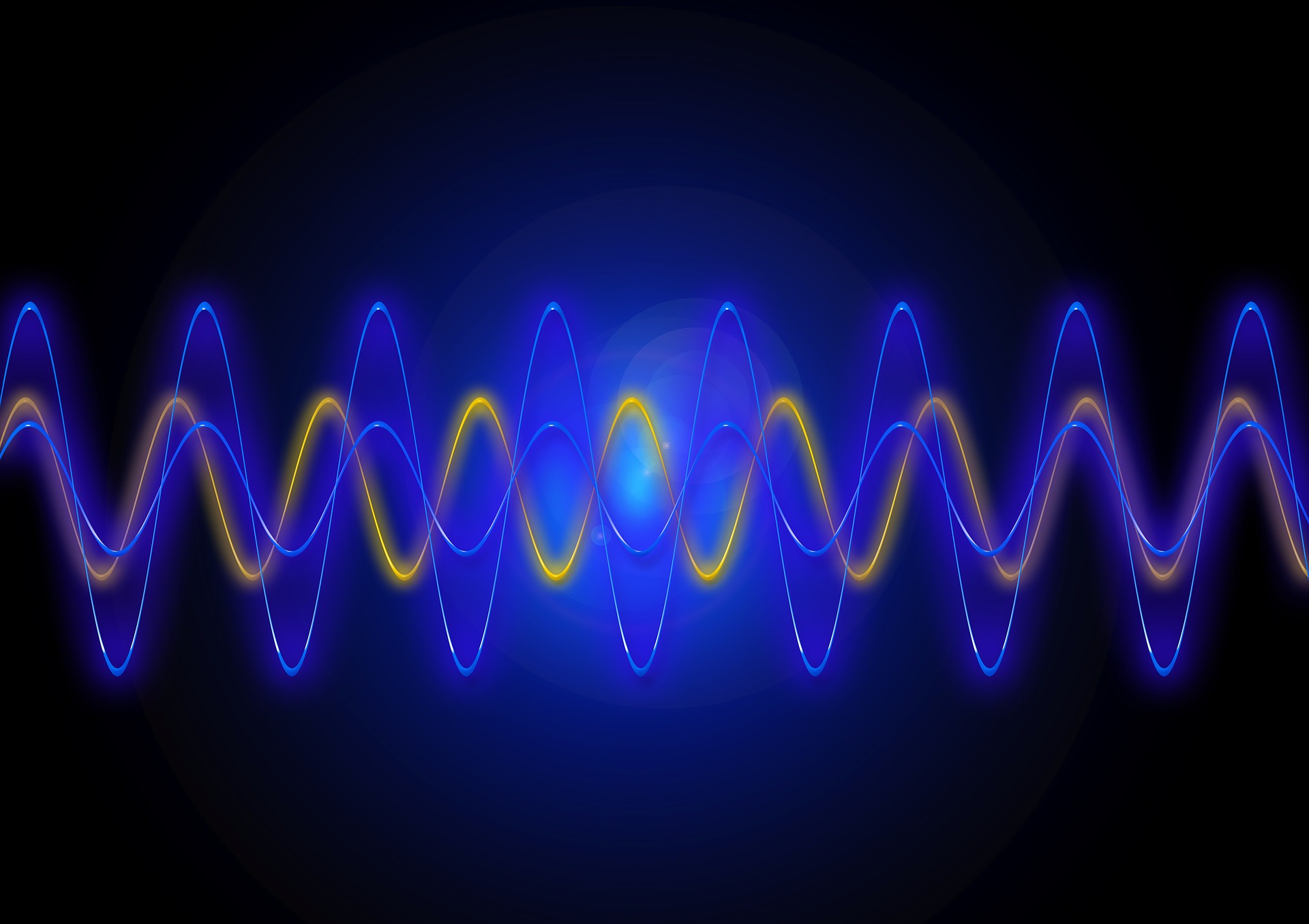
- DOI:
- 10.60864/7d6c-ry63
- Citation Author(s):
- Submitted by:
- Anonymous Submission
- Last updated:
- 6 February 2025 - 4:02am
- Document Type:
- Supplementary material
- Categories:
- Keywords:
- Log in to post comments
In image recognition, knowledge distillation is a valuable approach to train a compact model with high accuracy by exploiting outputs of a highly accurate large model as correct labels. In knowledge distillation, studies have shown the usefulness of data with high entropy output generated by image mix data augmentation techniques. Other strategies such as curriculum learning have also been proposed to improve model generalization by the control of the difficulty of training data over the learning process. In this paper, we explore the relationship between the learning process and data characteristics, focusing on the entropy of output distribution and learning difficulty in knowledge distillation. To validate this relationship, we propose a method to readily generate data that yields high entropy output and adjust the degree of learning difficulty by controlling bounds and a sampling range for mix ratios in mixing images between classes. In evaluation experiments using multiple datasets, our proposed method outperformed conventional approaches in terms of accuracy. This supplementary material gives details of experimental results.