Documents
Supplementary Material
MAP-YNET: LEARNING FROM FOUNDATION MODELS FOR REAL-TIME, MULTI-TASK SCENE PERCEPTION
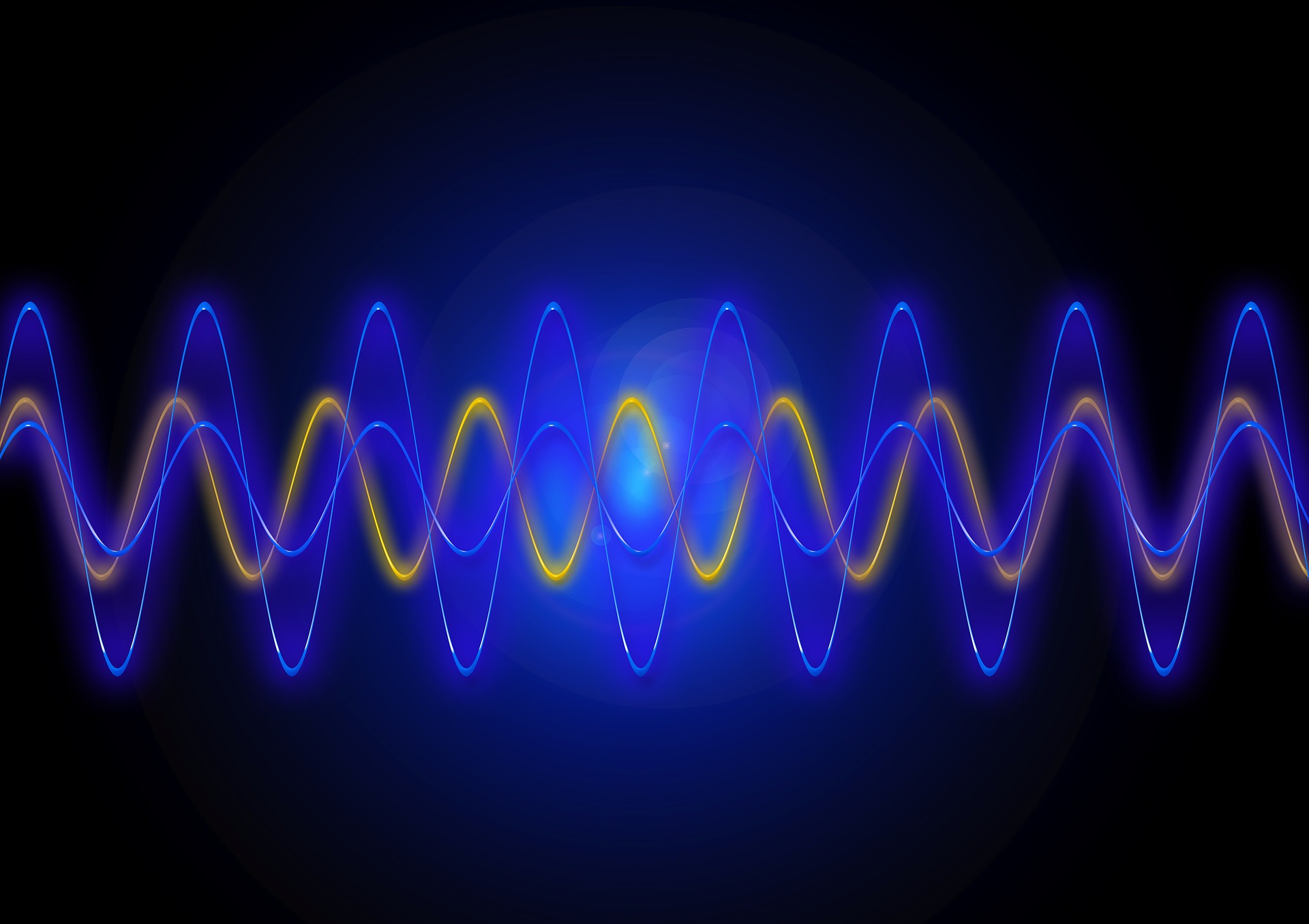
- Citation Author(s):
- Submitted by:
- Ammar Qammaz
- Last updated:
- 4 February 2025 - 8:26am
- Document Type:
- Supplementary Material
- Categories:
- Log in to post comments
This is video and qualitative supplementary material for ICIP 2025
We present MAP-YNet, a Y-shaped neural network architecture designed for real-time multi-task learning on RGB images. MAP-YNet simultaneously predicts depth, surface normals, human pose, semantic segmentation, and generates multi-label captions via a single network evaluation. To achieve this, we adopt a multiteacher, single student training paradigm, where task-specific foundation models supervise the network’s learning, enabling it to distill their capabil-
ities into an architecture suitable for real-time applications. MAP-YNet exhibits strong generalization, simplicity, and computational efficiency, making it ideal for robotics and other practical scenarios. To support future research, we will release our code publicly