Documents
Supplementary figures
Highly Precise Motion Transitions Detection in Untrimmed Sports Videos Using Spatio-Temporal Graph Embeddings
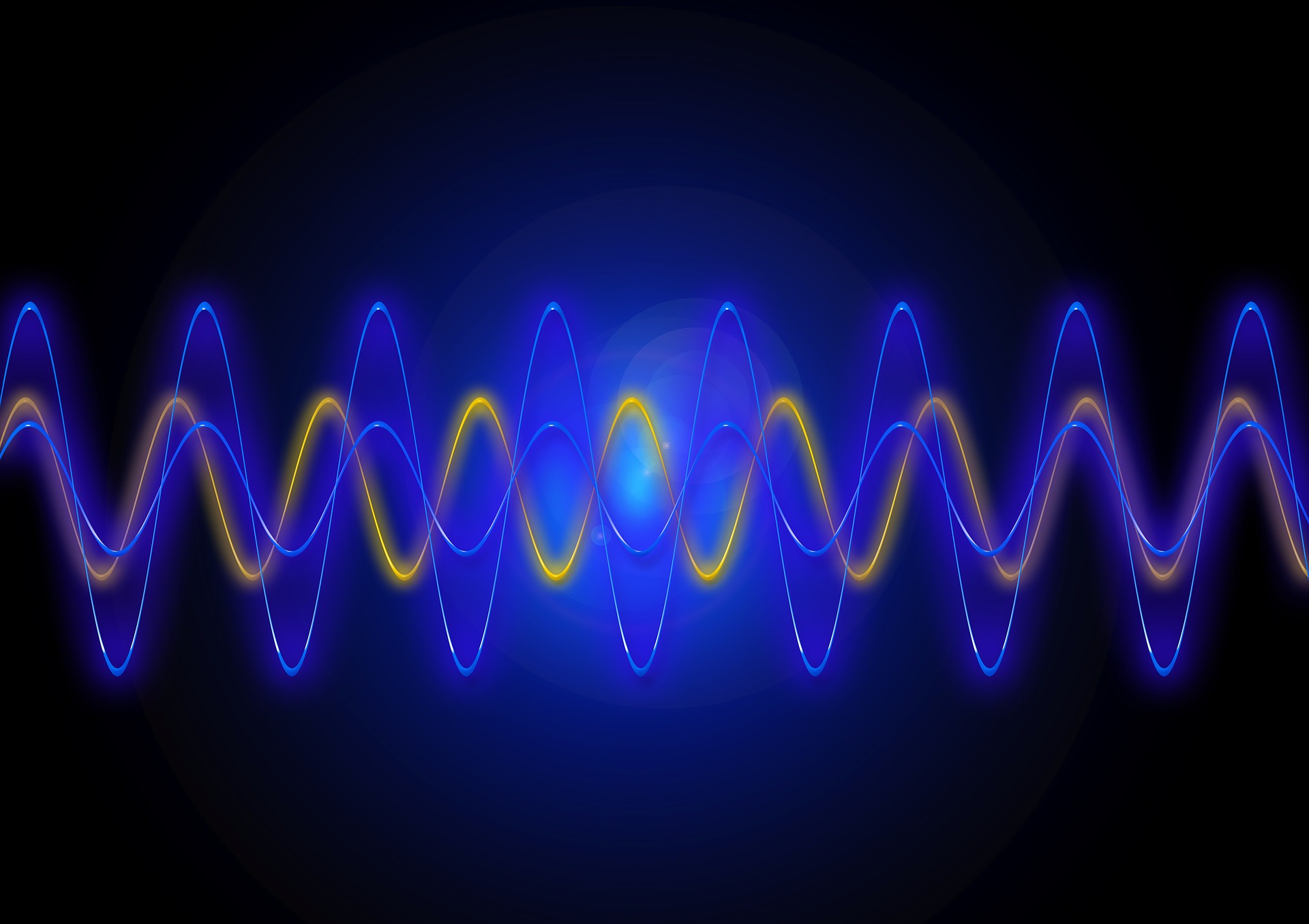
- Citation Author(s):
- Submitted by:
- Vipul Baghel
- Last updated:
- 5 February 2025 - 5:23pm
- Document Type:
- Supplementary figures
- Categories:
- Log in to post comments
Fine-grained action localization in untrimmed sports videos is a challenging task, as motion transitions are subtle and occur within short time spans. Traditional supervised and weakly supervised methods require extensive labeled data, making them less scalable and generalizable. To address these challenges, we propose an unsupervised skeleton-based action localization pipeline that detects fine-grained action boundaries using spatio-temporal graph embeddings. Our approach involves pre-training an Attention-based Spatio-Temporal Graph Convolutional Network (ASTGCN) on a blockwise partitioned pose-sequence to pose-sequence denoising task, enabling the model to learn motion dynamics in an unsupervised manner. During inference, we introduce an Action Dynamics Metric (ADM), computed from ASTGCN-derived embeddings, to detect motion transitions based on inflection points in the curvature of the ADM sequence. Experiments conducted on the DSV Diving dataset demonstrate that our unsupervised method achieves mAP of 29.09 ms and IoU of 0.57, which is comparable to state-of-the-art supervised methods. Additionally, our approach generalizes well to in-the-wild diving videos without requiring labeled data, proving its robustness and scalability for real-world applications.