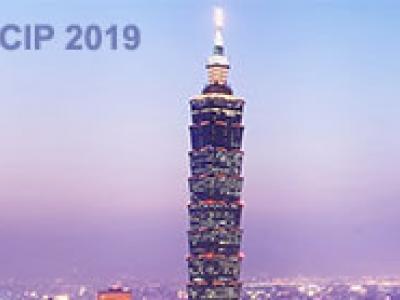
- Read more about Towards Unsupervised Single Image Dehazing with Deep Learning
- Log in to post comments
Deep learning computation is often used in single-image dehazing techniques for outdoor vision systems. Its development is restricted by the difficulties in providing a training set of degraded and ground-truth image pairs. In this paper, we develop a novel model that utilizes cycle generative adversarial network through unsupervised learning to effectively remove the requirement of a haze/depth data set. Qualitative and quantitative experiments demonstrated that the proposed model outperforms existing state-of-the-art dehazing models when tested on both synthetic and real haze images.
- Categories: