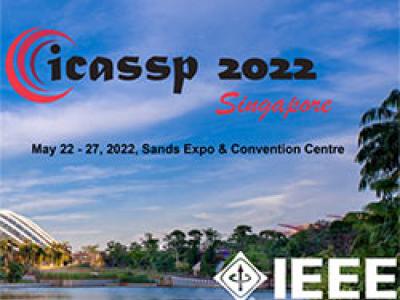
- Read more about Phonotactic Language Recognition using a Universal Phoneme Recognizer and a Transformer Architecture
- 1 comment
- Log in to post comments
In this paper, we describe a phonotactic language recognition model that effectively manages long and short n-gram input sequences to learn contextual phonotacticbased vector embeddings. Our approach uses a transformerbased encoder that integrates a sliding window attention to attempt finding discriminative short and long cooccurrences of language dependent n-gram phonetic units. We then evaluate and compare the use of different phoneme recognizers (Brno and Allosaurus) and sub-unit tokenizers to help select the more discriminative n-grams.
Poster.pdf

- Categories: