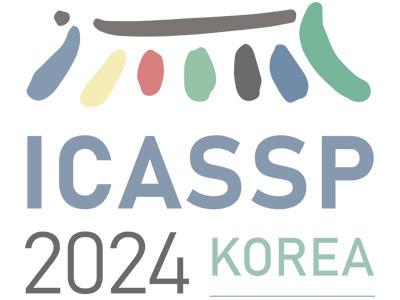
- Read more about ENHANCING NOISY LABEL LEARNING VIA UNSUPERVISED CONTRASTIVE LOSS WITH LABEL CORRECTION BASED ON PRIOR KNOWLEDGE
- Log in to post comments
To alleviate the negative impacts of noisy labels, most of the noisy label learning (NLL) methods dynamically divide the training data into two types, “clean samples” and “noisy samples”, in the training process. However, the conventional selection of clean samples heavily depends on the features learned in the early stages of training, making it difficult to guarantee the cleanliness of the selected samples in scenarios where the noise ratio is high.
- Categories: