- Read more about HOW VIDEO OBJECT TRACKING IS AFFECTED BY IN-CAPTURE DISTORTIONS?
- Log in to post comments
Video Object Tracking -VOT- in realistic scenarios is a difficult task. Image factors such as occlusion, clutter, confusion, object shape, and zooming, among others, have an impact on video tracker methods performance. While these conditions do affect trackers performance, there is not a clear distinction between the scene content challenges like occlusion and clutter, against challenges due to distortions generated by capture, compression, processing, and transmission of videos. This paper is concerned with the latter interpretation of quality as it affects VOT performance.
- Categories:
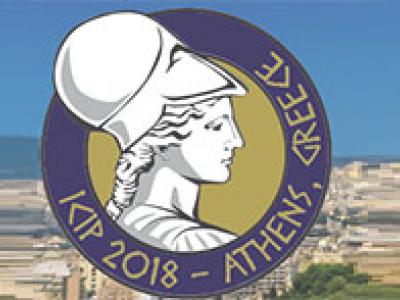
- Read more about DEEP MATCH TRACKER: CLASSIFYING WHEN DISSIMILAR, SIMILARITY MATCHING WHEN NOT
- Log in to post comments
Visual tracking frameworks employing Convolutional Neural Networks (CNNs) have shown state-of-the-art performance due to their hierarchical feature representation. While classification and update based deep neural net tracking have shown good performance in terms of accuracy, they have poor tracking speed. On the other hand, recent matching based techniques using CNNs show higher than real-time speed in tracking but this speed is achieved at a considerably lower accuracy.
- Categories:
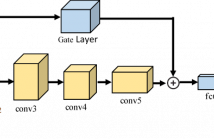
- Read more about Gate connected Convolutional Neural Network for Object Tracking
- Log in to post comments
Convolutional neural networks (CNNs) have been employed in visual tracking due to their rich levels of feature representation. While the learning capability of a CNN increases with its depth, unfortunately spatial information is diluted in deeper layers which hinders its important ability to localise targets. To successfully manage this trade-off, we propose a novel residual network based gating CNN architecture for object tracking. Our deep model connects the front and bottom convolutional features with a gate layer.
- Categories:
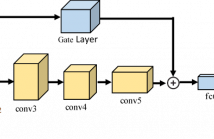
- Read more about Gate connected Convolutional Neural Network for Object Tracking
- Log in to post comments
Convolutional neural networks (CNNs) have been employed in visual tracking due to their rich levels of feature representation. While the learning capability of a CNN increases with its depth, unfortunately spatial information is diluted in deeper layers which hinders its important ability to localise targets. To successfully manage this trade-off, we propose a novel residual network based gating CNN architecture for object tracking. Our deep model connects the front and bottom convolutional features with a gate layer.
poster_ICIP.pdf

- Categories:
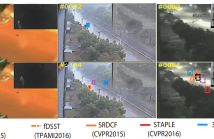
Visual context has formed a robust stimulation for visual perception. Spatio-temporal context in existing trackers sometimes shows weak reliability in visible light videos with poor quality. Supplemented by the infrared perception, this work exploits the role of visual context in tracking in a spatial-sequential-spectral view, by which to excavate dominance of different contexts in various scenarios.
- Categories: