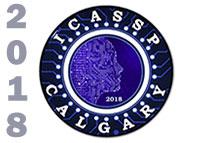
ICASSP is the world’s largest and most comprehensive technical conference focused on signal processing and its applications. The 2019 conference will feature world-class presentations by internationally renowned speakers, cutting-edge session topics and provide a fantastic opportunity to network with like-minded professionals from around the world. Visit website.
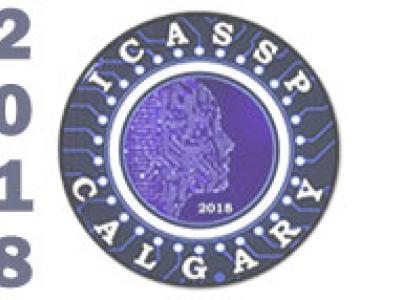
- Read more about Leveraging LSTM Models for Overlap Detection in Multi-Party Meetings
- Log in to post comments
The detection of overlapping speech segments is of key importance in speech applications involving analysis of multi-party conversations. The detection problem is challenging because overlapping speech segments are typically captured as short speech utterances far-field microphone recordings. In this paper, we propose detection of overlap segments using a neural network architecture consisting of long-short term memory (LSTM) models. The neural network architecture learns the presence of overlap in speech by identifying the spectrotemporal structure of overlapping speech segments.
- Categories:
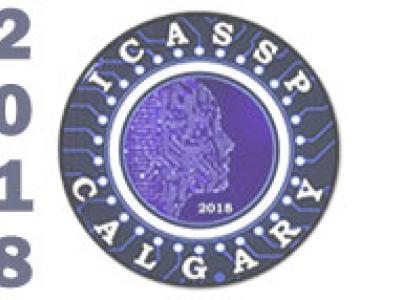
- Read more about END-TO-END DYNAMIC QUERY MEMORY NETWORK FOR ENTITY-VALUE INDEPENDENT TASK-ORIENTED DIALOG
- Log in to post comments
icassp18-poster.pdf

- Categories:
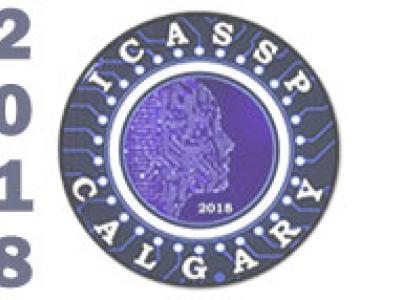
- Read more about MONOPHONE-BASED BACKGROUND MODELING FOR TWO-STAGE ON-DEVICE WAKE WORD DETECTION
- Log in to post comments
- Categories:
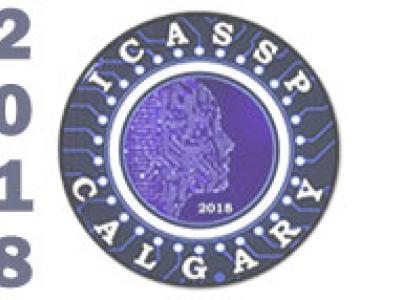
- Read more about QUICKEST CHANGE-POINT DETECTION OVER MULTIPLE DATA STREAMS VIA SEQUENTIAL OBSERVATIONS
- Log in to post comments
- Categories:
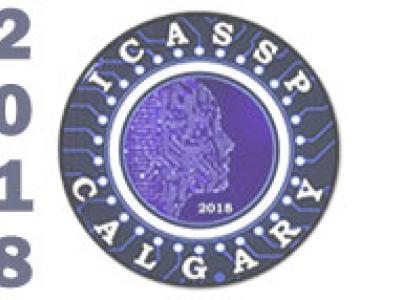
- Read more about The Landscape of Non-convex Quadratic Feasibility
- Log in to post comments
Motivated by applications such as ordinal embedding and collaborative ranking, we formulate homogeneous quadratic feasibility as an unconstrained, non-convex minimization problem. Our work aims to understand the landscape (local minimizers and global minimizers) of the non-convex objective, which corresponds to hinge losses arising from quadratic constraints. Under certain assumptions, we give necessary conditions for non-global, local minimizers of our objective and additionally show that in two dimensions, every local minimizer is a global minimizer.
ICASSP_v4.pdf

- Categories:
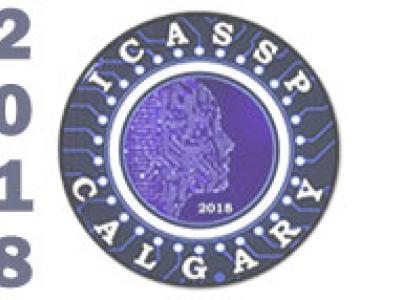
- Read more about Semi-blind Channel Estimation in Massive MIMO Systems with Different Priors on Data Symbols
- Log in to post comments
This paper investigates semi-blind channel estimation in massive multiple-input multiple-output (MIMO) systems using different priors on data symbols. We derive two tractable expectation-maximization (EM) based channel estimation algorithms; one based on a Gaussian prior and the other one based on a Gaussian mixture model (GMM) for the unknown data symbols. The numerical results show that the semi-blind estimation schemes provide better channel estimates compared with the estimation based on training sequences only.
- Categories:
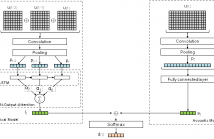
- Read more about Lexico-acoustic Neural-based Models for Dialog Act Classification
- Log in to post comments
Recent works have proposed neural models for dialog act classification in spoken dialogs.
However, they have not explored the role and the usefulness of acoustic information.
We propose a neural model that processes both lexical and acoustic features for classification.
Our results on two benchmark datasets reveal that acoustic features are helpful in improving the overall accuracy.
- Categories:
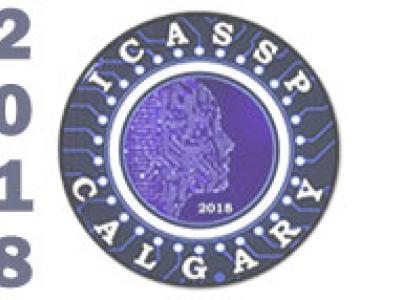
- Read more about Robust Distributed Gradient Descent with Arbitrary Number of Byzantine Attackers
- Log in to post comments
Due to the grow of modern dataset size and the desire to harness computing power of multiple machines, there is a recent surge of interest in the design of distributed machine learning algorithms. However, distributed algorithms are sensitive to Byzantine attackers who can send falsified data to prevent the convergence of algorithms or lead the algorithms to converge to value of the attackers' choice. Our novel algorithm can deal with an arbitrary number of Byzantine attackers.
- Categories:
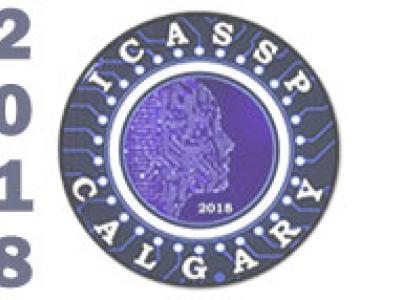
- Read more about Probability Reweighting in Social Learning: Optimality and Suboptimality
- Log in to post comments
This work explores sequential Bayesian binary hypothesis testing in the social learning setup under expertise diversity. We consider a two-agent (say advisor-learner) sequential binary hypothesis test where the learner infers the hypothesis based on the decision of the advisor, a prior private signal, and individual belief. In addition, the agents have varying expertise, in terms of the noise variance in the private signal.
- Categories: