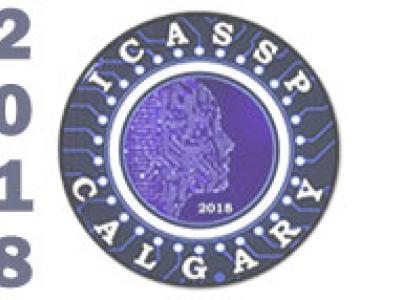
- Read more about PARAMETER ESTIMATION OF HEAVY-TAILED RANDOM WALK MODEL FROM INCOMPLETE DATA
- Log in to post comments
- Categories:
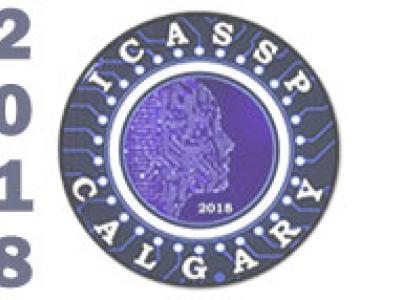
- Read more about PARAMETER ESTIMATION OF HEAVY-TAILED RANDOM WALK MODEL FROM INCOMPLETE DATA
- Log in to post comments
- Categories:
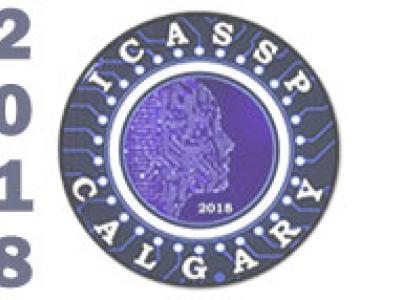
We extend the classical joint problem of signal demixing, blind deconvolution,
and filter identification to the realm of graphs. The model is that
each mixing signal is generated by a sparse input diffused via a graph filter.
Then, the sum of diffused signals is observed. We identify and address
two problems: 1) each sparse input is diffused in a different graph; and 2)
all signals are diffused in the same graph. These tasks amount to finding
the collections of sources and filter coefficients producing the observation.
- Categories:
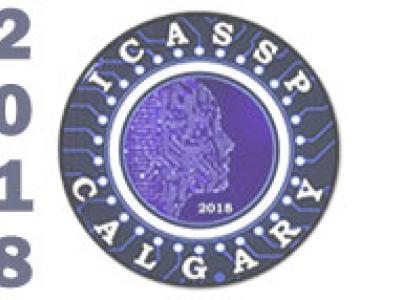
Henze-Penrose divergence is a non-parametric divergence measure that can be used to estimate a bound on the Bayes error in a binary classification problem. In this paper, we show that a cross- match statistic based on optimal weighted matching can be used to directly estimate Henze-Penrose divergence. Unlike an earlier approach based on the Friedman-Rafsky minimal spanning tree statistic, the proposed method is dimension-independent. The new approach is evaluated using simulation and applied to real datasets to obtain Bayes error estimates.
icassp2018.pdf

- Categories:
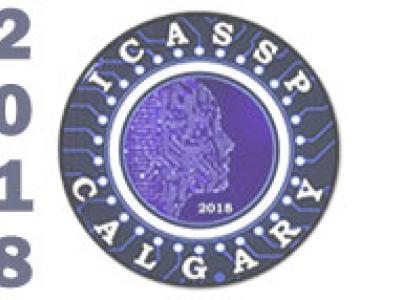
- Read more about Matching Pursuit Based Convolutional Sparse Coding
- Log in to post comments
Sparse coding techniques for image processing traditionally rely on processing small overlapping patches separately followed by averaging. This has the disadvantage that the reconstructed image no longer obeys the sparsity prior used in the processing. For this purpose convolutional sparse coding has been introduced, where a shift-invariant dictionary is used and the sparsity of the recovered image is maintained. Most such strategies target the $\ell_0$ ``norm'' of the whole image, which may create an imbalanced sparsity across various regions in the image.
- Categories:
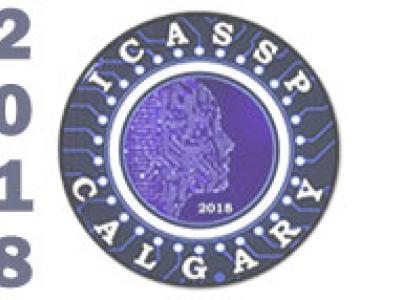
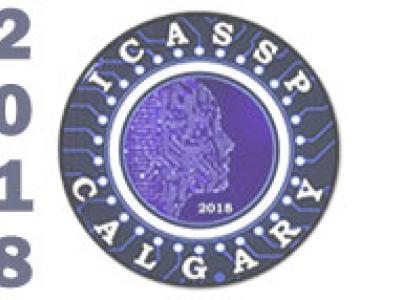
- Read more about TWO-STAGE IDENTIFICATION OF LOCALLY STATIONARY AUTOREGRESSIVE PROCESSES AND ITS APPLICATION TO THE PARAMETRIC SPECTRUM ESTIMATION
- Log in to post comments
The problem of identification of a nonstationary autoregressive process
with unknown, and possibly time-varying, rate of parameter
changes, is considered and solved using the parallel estimation approach.
The proposed two-stage estimation scheme, which combines
the local estimation approach with the basis function one, offers
both quantitative and qualitative improvements compared with
the currently used single-stage methods.
- Categories:
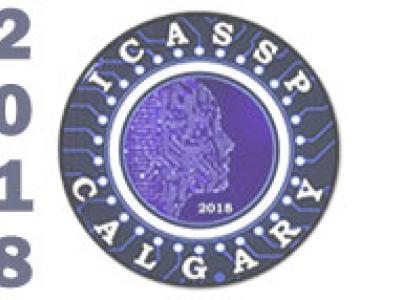
- Read more about COMPLEMENTARY COMPLEX-VALUED SPECTRUM FOR REAL-VALUED DATA: REAL TIME ESTIMATION OF THE PANORAMA THROUGH CIRCULARITY-PRESERVING DFT
- Log in to post comments
- Categories:
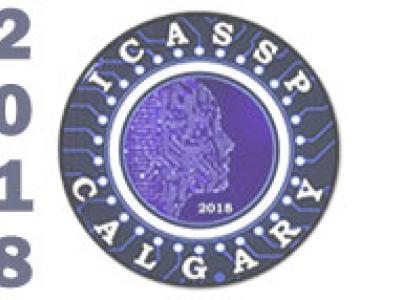
- Read more about On the Computability of System Approximations under Causality Constraints
- Log in to post comments
Approximating the transfer function of stable causal linear systems by a basis expansion is a common task in signal- and system theory. This paper characterizes a scale of signal spaces, containing stable causal transfer functions, with a very simple basis (the Fourier basis) but which is not computable. Thus it is not possible to determine the coefficients of this basis expansion on any digital computer such that the approximation converges to the desired function.
- Categories: