Documents
Presentation Slides
Beyond the Limit of Weight-Sharing: Pioneering Space-Evolving NAS with Large Language Models
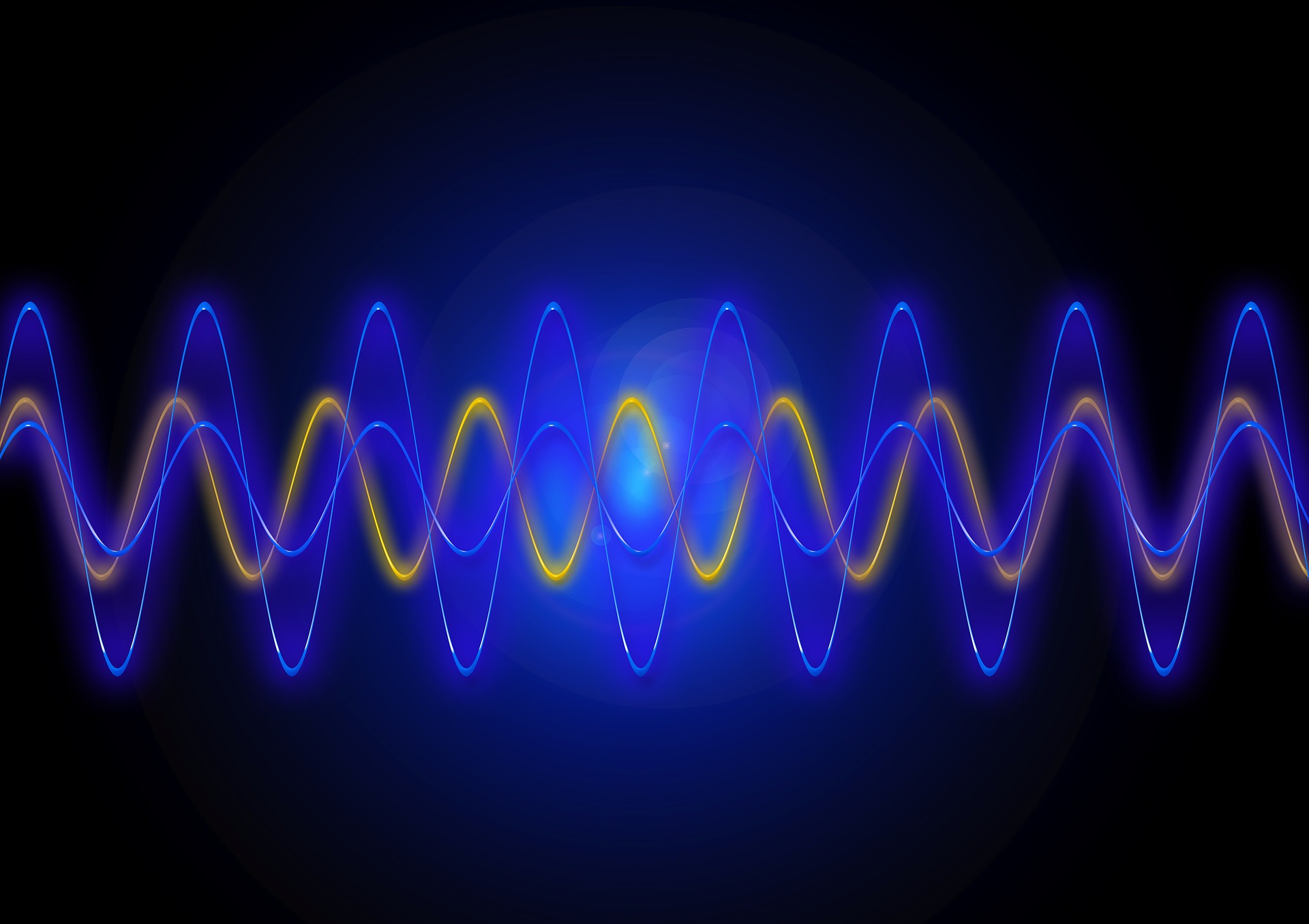
- Citation Author(s):
- Submitted by:
- Xiu Su
- Last updated:
- 12 April 2024 - 8:57am
- Document Type:
- Presentation Slides
- Categories:
- Log in to post comments
Large language models (LLMs) offer impressive performance across diverse fields, but their increasing complexity raises both design costs and the need for specialized expertise. These challenges are intensified for Neural Architecture Search (NAS) methods reliant on weight-sharing techniques. This paper introduces GNAS, a new NAS method that boosts the search process with the aid of LLMs for efficient model discovery. With insights from existing architectures, GNAS swiftly identifies superior models that can adapt to changing resource constraints. We provide a mathematical framework to facilitate the transfer of knowledge across different model sizes, thereby improving search efficiency. Our experiments conducted on ImageNet, NAS-Bench-Macro, and Channel-Bench-Macro confirm the effectiveness of GNAS across both CNN and Transformer architectures.
Comments
This is the presentation
This is the presentation slides of the paper titled "BEYOND THE LIMIT OF WEIGHT-SHARING: PIONEERING SPACE-EVOLVING NAS WITH LARGE LANGUAGE MODELS".