Documents
Presentation Slides
SURFACE EMG-BASED HAND GESTURE RECOGNITION VIA DILATED CONVOLUTIONAL NEURAL NETWORKS
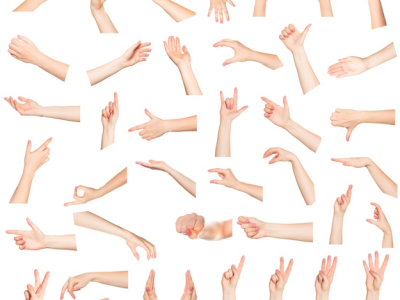
- Citation Author(s):
- Submitted by:
- Elahe Rahimian ...
- Last updated:
- 7 November 2019 - 10:35am
- Document Type:
- Presentation Slides
- Document Year:
- 2019
- Event:
- Presenters:
- Elahe Rahimian
- Paper Code:
- 1570567928
- Categories:
- Log in to post comments
The recent evolution of Artificial Intelligence (AI) and deep learning models coupled with advancements of assistive robotic systems have shown great potential in significantly improving myoelectric control of prosthetic devices. In this regard, the paper proposes a novel deep-learning-based architecture for processing surface Electromyography (sEMG) signals to classify and recognize upper-limb hand gestures via incorporation of dilated causal convolutions. The proposed approach has the potential to significantly improve the overall recognition accuracy due to the specific design of the convolutional layers. By using dilated causal convolutions which gradually increases the receptive field of the network, and by applying Conv1D, the proposed architecture eliminates the need for readjustment of the input sequences and inherently takes into account the hidden temporal correlations existing among the available set of sEMG sequences. Contrary to recent hybrid (RNN-CNN) solutions, the proposed architecture neither uses recurrent units nor 2-dimensional convolutions. The publically-accessible NinaPro DB2 dataset is utilized for training and evaluation of the proposed network. Through an extensive set of experiments, it is observed that the proposed architecture significantly outperforms its state-of-the-art counterparts and provides an average 8.71% performance improvement.
Comments
Hand Gesture Recognition
Hand Gesture Recognition