Documents
Presentation Slides
Acoustic Scene Classification for Mismatched Recording Devices Using Heated-Up Softmax and Spectrum Correction
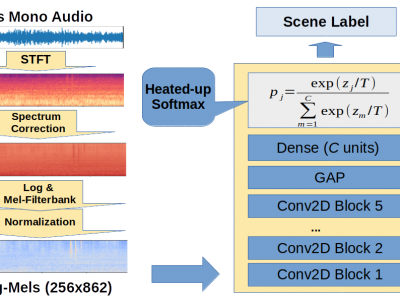
- Citation Author(s):
- Submitted by:
- Truc Nguyen
- Last updated:
- 13 May 2020 - 5:52pm
- Document Type:
- Presentation Slides
- Document Year:
- 2020
- Event:
- Presenters:
- Truc Nguyen
- Paper Code:
- 10.1109/ICASSP40776.2020.9053582
- Categories:
- Log in to post comments
Deep neural networks (DNNs) are successful in applications with matching inference and training distributions. In realworld scenarios, DNNs have to cope with truly new data samples during inference, potentially coming from a shifted data distribution. This usually causes a drop in performance. Acoustic scene classification (ASC) with different recording devices is one of this situation. Furthermore, an imbalance in quality and amount of data recorded by different devices causes severe challenges. In this paper, we introduce two calibration methods to tackle these challenges. In particular, we applied scaling of the features to deal with varying frequency response of the recording devices. Furthermore, to account for the shifted data distribution, a heated-up softmax is embedded to calibrate the predictions of the model. We use robust and resource-efficient models, and show the efficiency of heated-up softmax. Our ASC system reaches state-of-the-art performance on the development set of DCASE challenge 2019 task 1B with only ~70K parameters. It achieves 70.1% average classification accuracy for device B and device C. It performs on par with the best single model system of the DCASE 2019 challenge and outperforms the baseline system by 28.7% (absolute).