Documents
Presentation Slides
Active Regression with Compressive Sensing based Outlier Mitigation for both Small and Large Outliers
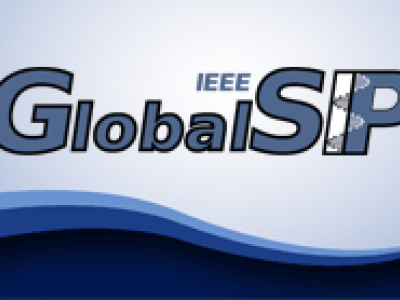
- Citation Author(s):
- Submitted by:
- Jian Zheng
- Last updated:
- 7 December 2016 - 3:00pm
- Document Type:
- Presentation Slides
- Document Year:
- 2016
- Event:
- Presenters:
- Jian Zheng
- Paper Code:
- 1231
- Categories:
- Log in to post comments
In this paper, a new active learning scheme is proposed for linear
regression problems with the objective of resolving the insufficient
training data problem and the unreliable training data labeling prob-
lem. A pool-based active regression technique is applied to select the
optimal training data to label from the overall data pool. Then, com-
pressive sensing is exploited to remove labeling errors if the errors
are sparse and have large enough magnitudes, which are called large
outliers. Next, in order to mitigate the non-sparse labeling errors that
have relatively small magnitudes, which are called small outliers, a
new technique is developed to convert them back into sparse large
outliers. With both artificial and real data sets, extensive simulations
are conducted to verify the robustness of the proposed scheme in
training data selection and outlier suppression